NCBI Bookshelf. A service of the National Library of Medicine, National Institutes of Health.
Duarte A, Mebrahtu T, Goncalves PS, et al. Adalimumab, etanercept and ustekinumab for treating plaque psoriasis in children and young people: systematic review and economic evaluation. Southampton (UK): NIHR Journals Library; 2017 Nov. (Health Technology Assessment, No. 21.64.)
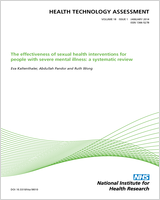
Adalimumab, etanercept and ustekinumab for treating plaque psoriasis in children and young people: systematic review and economic evaluation.
Show detailsBayesian NMA was conducted to pool trial results. NMA models were programmed in WinBUGS software (version 1.4.3) using a Bayesian statistical framework. WinBUGS is a Bayesian analysis software tool that, through the use of Gibbs sampling (a Markov chain Monte Carlo method), evaluates posterior distributions for the parameters of interest given likelihood functions derived from data and prior probabilities. Fixed- and random-effects models were evaluated. Model selection was determined by model fit statistics (i.e. DIC and total residual deviance) to identify the best model choice. Treatment effects were expressed in relation to placebo. Uninformative priors were used throughout. The Bayesian NMA for PASI utilised a framework of analysis that evaluated the probability of PASI responses in different categories of PASI thresholds (50, 75 and 90) within a single model. The analyses followed the principles outlined in the NICE Decision Support Unit (DSU).95 The single synthesis multinomial model with a probit link is recommended by the NICE DSU and assumes that there is an underlying continuous variable that has been categorised by specifying the cut-off points. It assumes also that the treatment effect is the same regardless of the different cut-off points in each trial. All PASI response models were run for 10,000 iterations after a burn-in of 20,000 on two chains. Synthesis model results provide pooled probabilities of achieving PASI 50, 75 and 90 responses for each treatment of interest, alongside a measure of uncertainty, that is, the 95% CrI. In brief, trials report rikj, the number of patients in arm k of trial i belonging to different, mutually exclusive categories j = 1, 2, 3, where these categories represent the different thresholds of PASI score (e.g. 50%, 75% or 90% improvement). The responses for each arm k of trial i in category j follow a multinomial distribution:
which has been parameterised as a series of conditional binomial distributions, with parameters of interest the probabilities, pikj, that a patient in arm k (k = 1, 2, 3) of trial i (i = 1,. . . . .,I) belongs to category j (j = 1, 2, 3). A probit link function was used, the inverse of the normal cumulative distribution function Φ, to define pikj as a function of a set of threshold values, zj. The threshold values (estimated within the model) are such that the probability that the standard normal (the probit score) will take a value less than or equal to z1 will reflect the probability of obtaining a PASI response lower than 50%, that is, 1 – PASI50. The probability that the standard normal will take a value less than or equal to z2 will reflect the probability of obtaining a PASI response lower than 75%, that is, 1 – PASI75 and, analogously, evaluating Φ at z3 will approximate 1 – PASI90. Placebo and treatments are assumed to shift the mean of the distribution. This means that the pooled effect of taking the experimental treatment instead of the control is to change the probit score (or z-score) of the control arm by di,1 standard deviations. Therefore, the model is written as:
We assumed that the baselines, µi, were trial specific (i.e. unconstrained – except for model 1b) and were given a non-informative prior. A non-informative prior was assigned to the treatment effects parameter (δt). A uniform prior was assigned to the parameter zj.
Alternative assumptions were tested in two analyses. The first assumed a meta-regression for placebo effects (model 2a). In a second analysis we explored the impact on treatment effects of adjusting for age, that is, explicitly modelling children and young people and adult subgroups (model 2b). The key assumptions implemented for PASI responses in the models and detailed coding of the models are presented in Table 99. The preferred model was used to evaluate the estimated probability of achieving PASI 50, 75 and 90 responses on treatment t, using:
TABLE 99
Description of models and underlying assumptions for PASI response
where µi1 is the baseline effects, i is the studies and 1 = placebo, NS is the number of studies and B is the common regression (slope) coefficient relating to the treatment*age interaction, which is assumed to be identical for all treatments. This is a strong assumption but, because of only increasing the number of parameters in the model by 1, is the least data demanding. Other interaction assumptions were tested (i.e. independent and exchangeable)138 but the model was unable to appropriately estimate all parameters.
We adopted the WinBUGS code presented in DSU2239 for the analysis, although we identified that the model was not specifying the z-score correctly in the linear predictor specification when the first category of the response data (in this case PASI 50) was missing. A correction was made to incorporate the correct specification for the z-score in the linear predictor specification.
WinBUGS code for the preferred model
- Evidence synthesis modelling, software and WinBUGS code - Adalimumab, etanercept...Evidence synthesis modelling, software and WinBUGS code - Adalimumab, etanercept and ustekinumab for treating plaque psoriasis in children and young people: systematic review and economic evaluation
- Genome Links for Protein (Select 1937369960) (1)Genome
- Related DataSets for GEO Profiles (Select 56815337) (1)GEO DataSets
- Dicer deficient embryonic stem cell response to miR-290 transfectionDicer deficient embryonic stem cell response to miR-290 transfectionAccession: GDS3430GEO DataSets
Your browsing activity is empty.
Activity recording is turned off.
See more...