Included under terms of UK Non-commercial Government License.
NCBI Bookshelf. A service of the National Library of Medicine, National Institutes of Health.
Davis S, Martyn-St James M, Sanderson J, et al. A systematic review and economic evaluation of bisphosphonates for the prevention of fragility fractures. Southampton (UK): NIHR Journals Library; 2016 Oct. (Health Technology Assessment, No. 20.78.)
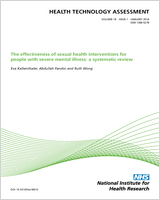
A systematic review and economic evaluation of bisphosphonates for the prevention of fragility fractures.
Show detailsSystematic review of existing cost-effectiveness evidence
Methods
The review of the published evidence surrounding the cost-effectiveness of bisphosphonates in the patient groups eligible for risk assessment within CG14616 was started by analysing the likely quantity of evidence available. A published systematic review by Müller et al.136 included cost-effectiveness studies of screen-and-treat strategies for preventing osteoporotic fractures published between January 2006 and November 2011. Of the 24 papers included by Müller et al.,136 22 examined the cost-effectiveness of bisphosphonates. However, only seven of these considered a UK setting.137–143 Given the large number of published articles identified from this single systematic review, it was decided to limit the review to those papers reporting cost-effectiveness analyses for a UK setting as they would be more applicable to the decision problem defined in Chapter 2. None of the consultee submissions contained a de novo economic evaluation, so the review of existing cost-effectiveness evidence is limited to published sources.
Identification of studies
A comprehensive search was undertaken until 26 September 2014 to identify papers published in 2006 or later that evaluated the cost-effectiveness of alendronic acid, risedronic acid, oral ibandronic acid, i.v. ibandronic acid or zoledronic acid in any of the patient groups eligible for risk assessment within CG146.16 Subject headings and keywords for ‘osteoporosis’ were combined with each of the named interventions and an economics search filter. The search strategy is provided in Appendix 1.
The following databases were searched:
- MEDLINE In-Process & Other Non-Indexed Citations and MEDLINE (via Ovid) 2006 to 23 September 2014
- EMBASE (via Ovid) 2006 to 23 September 2014
- Cochrane Database of Systematic Reviews (via Wiley Online Library) 2006 to 23 September 2014
- Database of Abstracts of Reviews of Effects (via Wiley Online Library) 2006 to 23 September 2014
- Health Technology Assessment Database (via Wiley Online Library) 2006 to 23 September 2014
- NHS Economic Evaluation Database (via Wiley Online Library) 2006 to 23 September 2014
- EconLit (via Ovid) 2006 to 23 September 2014
- Cumulative Index to Nursing and Allied Health Literature (via EBSCOhost) 2006 to 23 September 2014
- Science Citation Index Expanded (via Web of Science) 2006 to 23 September 2014
- Conference Proceedings Citation Index – Science (via Web of Science) 2006 to 23 September 2014
- Bioscience Information Service (via Web of Science) 2006 to 23 September 2014.
Published economic evaluations cited within the consultee submissions were cross-checked with those identified from the search.
Inclusions/exclusion criteria
Studies were included in the review if they reported full economic evaluations comparing alendronic acid, risedronic acid, oral ibandronic acid, i.v. ibandronic acid or zoledronic acid with each other or with no treatment. Studies were included if any of the population considered would be eligible for risk assessment within CG146.16 For example, studies on postmenopausal women were included whether or not they specified that the women had risk factors, as those aged > 65 years would be eligible for risk assessment under CG146 even without risk factors being present. Studies that did not assess outcomes using QALYs or report the incremental cost per QALY of alternative treatment strategies were excluded. Studies that did not assess the cost-effectiveness of bisphosphonates within a UK setting were also excluded, as discussed above. Studies that assessed the cost-effectiveness of treatment with bisphosphonates at non-licensed doses were also excluded, as were studies that used bisphosphonates for other indications, such as the treatment of Paget’s disease or metastatic bone disease. Studies published prior to 2006 were excluded on the basis that the estimates of cost-effectiveness from older published studies are unlikely to be directly applicable to the decision problem outlined in the scope because of the availability of generic bisphosphonates, which has reduced the price of bisphosphonates over recent years. Studies were included only if they were reported as full papers, with conference abstracts being excluded from the review as they present insufficient detail to allow for a rigorous assessment of study quality. Studies not reported in English were also excluded.
Review methods
The results of the economic searches were sifted by title and abstract by one reviewer (AR). The full papers of studies that potentially met the inclusion criteria were retrieved for further inspection. Studies included in the systematic review were examined to determine whether or not they met the NICE reference case.144 They were also critically appraised using the checklist published by Phillips et al.145
Results
The study selection process is summarised in the form of a PRISMA diagram96 in Figure 65.

FIGURE 65
Flow diagram of study selection process (adapted from PRISMA): cost-effectiveness review.
Quantity of evidence identified
The search identified 1058 unique articles, of which 1013 were excluded at the title and abstract stage. A further 37 were excluded at the full-paper stage, with the most common reasons being that they were conference abstracts presenting limited data. Table 50 in Appendix 7 provides the reasons for exclusion for those papers that were not excluded based on title or abstract. None of the consultee submissions identified any published analyses not already picked up through the systematic search.
Study characteristics
The characteristics of the included studies are summarised in Table 6. Six of the included studies137–141,146 were in postmenopausal women, with the remaining two being in populations with steroid-induced osteoporosis.142,143
TABLE 6
Characteristics of included studies: cost-effectiveness review
Three studies137–139 compared a single bisphosphonate with no treatment, one study140 compared multiple bisphosphonate strategies head to head and with no treatment, and four studies141–143,146 compared a strategy of bisphosphonates with no treatment without specifying the exact bisphosphonate used. All of the included studies assumed that treatment with bisphosphonates lasts 5 years.
Six studies137–140,142,146 used a Markov model framework, with four137–139,146 using a cohort-level modelling approach and two140,142 using a patient-level Markov simulation based on the same underlying model. The remaining two papers141,143 described an individual patient-based pharmacoeconomic model using patient-level data from two large GP record databases [General Practice Research Database (GPRD) and The Health Improvement Network].
Two studies140,142 explicitly reported using a NHS and Personal Social Services (PSS) perspective, while a further three studies137–139 reported using a health-care perspective and one reported a societal perspective.146 The remaining two studies141,143 did not explicitly report their perspective although many of the costs used were taken from Stevenson et al.140 which used a NHS and PSS perspective. Discounting consistent with the current NICE reference case144 (3.5% for both costs and QALYs) was applied in four of the studies,137–139,146 whereas alternative discounting at rates (6% for costs and 1.5% for QALYs) were used in the remaining four papers.140–143 The time horizon varied from 6 years to a lifetime horizon or age of 100 years.
Evidence sources used
The study conducted by Stevenson et al.140 was a systematic review of the literature to estimate the costs associated with osteoporotic fractures. The remaining studies used various sources including personal communication and pre-exiting literature, with two studies137,138 quoting the same source, Stevenson et al.147
For all published cost-effectiveness studies the costs of the pharmaceutical agents were ultimately taken from the appropriate version of the British National Formulary for their cost year. The costs of case finding, BMD testing and consultations with GPs were obtained from various sources including the appropriate versions of the NHS reference costs and the Unit Costs of Health and Social Care or were assumed.
Health-related quality of life was obtained using utility multipliers for fracture states taken from the literature. The studies use different categories of fracture, with hip fracture, vertebral fracture, forearm/wrist fracture and humerus fracture being the most common. One study had the additional categories of pelvic fracture, tibia fracture, clavicle, scapula or sternum fracture and rib fracture.142 Three studies further split hip fracture into hip fracture leading to nursing home admission and hip fracture not leading to nursing home admission.140,141,143 Seven studies split utility multipliers for fractures into those for the year of fracture and those in subsequent years.137–143 The remaining study split multipliers for fractures into those for the year of fracture and those in the year following fracture and those in subsequent years.146
The National Institute for Health and Care Excellence reference case
Both studies by van Staa et al.141,143 used data from a retrospective analysis of patient notes rather than RCT evidence, as required by the NICE reference case.144 These authors also reported results using a 10-year time horizon rather than the lifetime horizon, which is required by the NICE reference case. The study by Borgström et al.146 failed to meet the requirements of the NICE reference case as the RR reduction used in the study was based on an assumption involving the expected distribution of osteoporotic fractures dependent on age and the subsequent utility loss rather than on the evidence. Additionally, the study by Ström et al.139 failed to meet the requirements of the NICE reference case by using efficacy data from a single RCT, but the study did present the results of a sensitivity analysis using data from a published meta-analysis. Two papers, by Stevenson et al.140 and Kanis et al.,142 which used the same underlying model but applied it in two different populations, used differential discount rates of 6% for future costs and 1.5% for future benefits rather than 3.5% for both future costs and future benefits as required by the NICE reference case. However, Kanis et al.142 did report that using discount rates of 3.5% for both future costs and future benefits had only a minor effect on the results. In addition to the points above, none of the included studies compared all four bisphosphonates specified within the scope of this appraisal in a fully incremental analysis as required by the NICE reference case.
Quality of studies
The quality of the studies was generally good when appraised using the checklist published by Phillips et al.145 Responses for each individual study are provided in Table 7. Five of the studies met > 50% of the checklist criteria.137–140,142 The studies commonly performed badly on the questions related to internal and external consistency, with none of the models providing an adequate description of the quality assurance processes used to demonstrate internal validity and none demonstrating that the model had been calibrated against external data sources. All of the models assessed patient-level heterogeneity by running the model for subgroups of patients with different characteristics. However, none of the papers adequately addresses all types of uncertainty (structural, parameter, methodological). Three of the models139,140,142 assessed parameter uncertainty using analysis [probabilistic sensitivity analysis (PSA)], but in the other five cases this was either not done or not clearly reported. Only two of the studies140,142 adequately addressed the quality of the input data and there was limited discussion of the methods used to derive the utility weights applied in the model.
TABLE 7
Quality assessment of the included studies: cost-effectiveness
Study conclusions
All of the studies report a range of incremental cost-effectiveness ratios (ICERs) for patients with different characteristics. Patient age, BMD, the presence of prior fracture and the presence of other clinical risk factors all appear to have a significant influence on the ICER based on the included studies. The duration of treatment and the offset duration (the time over which the treatment still has an effect on fracture risk following discontinuation), as well as patient adherence to treatment, may have a lesser influence on the cost-effectiveness. Given that none of the studies used current prices for bisphosphonates and these have fallen substantially since the time these studies were published, further details on the ICERs are not reported.
Summary of existing cost-effectiveness evidence
Although a number of published studies were identified that assessed the cost-effectiveness of bisphosphonates, and the quality of those studies was generally good, none of the included studies compared all the bisphosphonate treatments specified within the scope of this appraisal in a fully incremental analysis as required by the NICE reference case.144 Furthermore, the cost of generic formulations of bisphosphonates has fallen since these studies were conducted. The results reported by these studies were, therefore, considered to have limited applicability to the decision problem described in Chapter 2. However, these studies were used as a source of model parameters and assumptions for the independent economic assessment described in Independent economic assessment.
Independent economic assessment
Modelling rationale and overview
A de novo economic analysis was considered necessary in order to properly address the decision problem outlined in the scope, as none of the economic evaluations identified in Systematic review of existing cost-effectiveness evidence compared all five bisphosphonate treatments specified within the scope of this appraisal in a fully incremental analysis as required by the NICE reference case.144
In the scope for this appraisal23 it was stated that this MTA would ‘develop the framework to link absolute fracture risk with intervention thresholds, based on cost effectiveness’. Therefore, in order to provide information that might inform intervention thresholds, expressed in terms of absolute risk, the aim of the de novo economic evaluation was to estimate the cost-effectiveness of the five bisphosphonates treatments compared with no treatment for patients at varying levels of absolute fracture risk. The overall population is those eligible for risk assessment under CG146,16 but it is divided into risk categories based on the estimates of fracture risk provided by the QFracture and FRAX risk assessment tools.
Discrete event simulation (DES) was used to estimate lifetime costs and QALYs for each bisphosphonate treatment strategy and a strategy of no treatment for a simulated cohort of patients with heterogeneous characteristics. The model was populated with effectiveness evidence from the systematic review and NMA described in Chapter 3. All other parameters were estimated from published sources. Evidence on the impact of fracture on HRQoL was identified from a systematic review. The published economic evaluations described in in Systematic review of existing cost-effectiveness evidence were used to identify other data sources that could be used to inform model parameters. A NHS and PSS perspective was taken and costs and benefits were discounted at 3.5% per annum. A brief summary of the modelling methodology and key data sources is provided in Table 8 alongside information of where further details can be found in Methods below.
TABLE 8
Overview of the modeling methodology and key data sources
Methods
Model structure
The model is a DES that simulates the clinical events occurring over the lifetimes of individual patients who are allowed to have heterogeneous characteristics. When designing the model structure, we constructed a conceptual model to explore the relationships between patient characteristics, absolute risks and cost-effectiveness, which is summarised in Figure 66 and discussed in more detail in the section Specifying the model population. Based on this conceptual model we anticipated that an unbiased estimate of the average cost-effectiveness for groups selected according to their level of absolute risk could only be obtained by calculating the mean cost-effectiveness across a population with heterogeneous characteristics. This is because we expected certain characteristics, such as age, which are not uniform across cohorts selected based on absolute risk, to have a non-linear relationship with cost-effectiveness. For example, age was expected to affect both life expectancy and the probability of a new admission to a residential care setting following fracture, both of which would alter the cost and QALY implications of fracture. Therefore, we decided to use a patient-level simulation approach in which the patient characteristics were allowed to vary stochastically in a manner that reflects our beliefs about their distribution within the general population. Having decided to use a patient-level simulation approach, we then decided that a DES approach would be more efficient than a patient-level state transition approach. This is because a DES approach updates the calculation of costs and benefits only when a patient experiences an event rather than making calculations for every model cycle. The cohort modelled included a substantial proportion of low-risk patients, as not all patients eligible for fracture risk assessment under CG14616 are at high risk of fracture. In a low-risk cohort it would be common for there to be no fracture events experienced during a patient’s lifetime. Calculating costs and QALYs every model cycle is much less efficient in low-risk populations than in high-risk populations, in which events may occur every few cycles. The main disadvantage of using a DES approach is that the risk factor tools (FRAX and QFracture), which are recommended for assessing fracture risk in CG146,16 provide estimates of the cumulative risk over a defined time frame (10 years for FRAX and 1–10 years for QFracture). In order to convert these estimates of absolute cumulative risk to time-to-event estimates, it was necessary to assume some functional form for event-free survival, and this required some additional data or assumptions regarding the hazard function.

FIGURE 66
Relationships assumed between individual risk factors and cost-effectiveness. COPD, chronic obstructive pulmonary disease; CVD, cardiovascular disease.
In general, within a DES model, patients’ experience as they progress through the model is determined by the events that occur rather than by the health states they occupy. Figure 67 shows the clinical events that can occur over a patient’s lifetime, with the arrows showing which events can occur following other events. (Note that this is not a state transition diagram, as patients do not reside in the state defined by the most recent event until the next event is experienced.) In our model, the main clinical events were fracture, death and new admission to residential care. Fractures at different sites were processed using separate fracture events for hip, wrist, and vertebral and proximal humerus. These are the sites most strongly associated with osteoporosis and these are the fracture sites included by both the QFracture and FRAX risk calculators. Fractures at additional sites (femoral shaft, humeral shaft, pelvis, scapula, clavicle, sternum, ribs, tibia and fibula) have been incorporated by increasing the incidence of these four event types rather than by adding additional competing events.

FIGURE 67
Clinical events that can occur during a patient’s lifetime in the DES.
Separate events are shown in Figure 67 for all-cause mortality and fracture-related deaths to show that fracture-related deaths can occur only following hip and vertebral fracture. However, in practice in the model code, a single event was used to process both all-cause mortality and fracture-related deaths. If a particular fracture was sampled to be fatal, then the time to death was set equal to the time of fracture plus an additional time assumed to be 3 months. At all other times, the time to death was determined by age- and sex-specific estimates for all-cause mortality from the general population. As the data provided by the life tables allowed only the year of death to be sampled and not the exact time point, we assumed that all deaths occurred exactly 6 months through the year in which death was sampled to occur. All-cause mortality estimates were not adjusted to remove deaths following fracture and, therefore, the model may have marginally overestimated the total mortality risk.
A schematic of the model logic used to implement the DES is provided in Figure 68. In a DES, the patient’s progress is driven by a list of times at which each event has been sampled to occur. The model steps forward from one event to the next and the list of event times can be updated when events are processed, to allow the patient’s event history to affect their future progress. In a DES no changes are made to the patient’s attributes between events. Therefore, dummy events were used to ensure that certain patient attributes were updated at times other than when experiencing a clinical event (death or fracture, or new admission to residential care home). For example, dummy events were used to recalculate fracture risks at the end of treatment and at the end of the period when treatment effect is assumed to reach zero. The time between the end of treatment and the end of any remaining treatment effect is called the fall-off period. If these two events occurred prior to 5 and 10 years, respectively, then additional dummy events are scheduled for 5 and 10 years to ensure that all patients have their risk updated at these time points. Dummy events were also used to allow the patient’s health utility values to be updated 1 year after a fracture event to allow the acute (< 1 year) and chronic (> 1 year) consequences of fracture to be incorporated separately. Finally, a time horizon event was also included to process final patient outcomes for those patients who do not die before reaching the age of 100 years. The individual’s risk of fracture is updated each time a clinical event, or dummy event, occurs. The model incorporates the following structural assumptions:

FIGURE 68
Schematic of the DES model.
- The maximum number of hip fractures that can be experienced is limited to one per bone with an additional limit of four vertebral fractures, four rib fractures and two pelvic fractures.
- There are no restrictions on the sequence of fractures that can be experienced.
- Death attributable to fracture occurs 3 months after fracture (see Mortality after hip fracture), with other fracture events possible during this period but no mortality from non-fracture-related causes.
- No further events can be experienced after death.
- A fracture event occurring < 1 year after a previous event supersedes the dummy event used to update patient attributes 1 year after fracture, thus reducing the acute period for the earlier fracture.
- Nursing home admission can only occur following fracture and, therefore, patients who are community dwelling at the start of the simulation do not transfer to nursing home care as they age, unless this is simulated to occur following a fracture.
Utility in the model is based on a combination of sex, age, fracture history and residential status (community dwelling or institutionalised). Every time an event occurs the patient’s utility value is updated and this utility value is used to calculate the QALYs accrued between one event and the next. Furthermore, when calculating the QALYs accrued between events an adjustment is made for age-related utility decrements over the intervening years so that the utility value applied does not remain artificially high when the time between events is long. This is done by assuming a linear fall in utility over the intervening years between events. The utility impact for each fracture type is separated into an acute utility multiplier applied in the first year after fracture and a chronic utility multiplier which is applied in all subsequent years. If more than one fracture has occurred then the chronic multiplier for each fracture is applied but no more than one acute utility multiplier is applied at any one time. A utility multiplier is also applied for institutional versus community living. Owing to the use of multipliers the absolute utility decrement for each subsequent fracture is smaller and the patient’s utility never falls to below zero. Patients who have a prior fracture (as defined by either the FRAX or QFracture risk calculators) at baseline have the chronic utility multiplier for that fracture type applied for rest of their lifetime.
Two types of costs are applied within the model to capture the consequences of fracture. Acute costs, which represent the cost of acute care such as hospitalisations, are assumed to occur at the time of the event and are applied for both fatal and non-fatal fractures. Chronic costs, which are used to represent the ongoing costs of care in the months and years after fracture, such as nursing home care or medication costs for chronic pain, are accrued gradually over the time period between events. The chronic cost is set to the maximum chronic cost for all fracture events experienced so far, with the maximum chronic cost for any individual being the cost for institutionalised patients. Drug costs are applied from the start of the simulation until the end of the treatment period and are assumed to accrue at a constant rate across time.
Death does not incur any additional costs within the model. For patients who suffer a fatal fracture, the full costs of acute care in the year following fracture are still incurred despite the reduced survival period of 3 months under the assumption that that majority of acute costs are incurred close the time of fracture.
Patients are assumed to stay in the same residential setting (community or institution) unless they experience a fracture event. So while some patients reside in an institutional setting at the start of the simulation, and this proportion is higher in older patients, no patients are simulated to move from the community into an institutional residential setting for reasons other than fracture. This may slightly overestimate the cost savings of preventing fractures, as in reality people may enter an institutional residential setting prior to a fracture occurring and, therefore, will not be at risk of incurring additional costs for residential care following fracture. However, this assumption avoids the need for regular events updating the patient’s residential status, which would reduce the computational efficiency of the DES approach.
The simulation for each individual ends when a fracture-related or non-fracture-related death occurs or when the time horizon is reached. The time horizon is set according to the patient’s starting age so that the simulation ends at age 100 years for all patients. This is because the all-cause mortality data are limited to patients aged ≤ 100 years. Costs and benefits have been discounted within the analysis at 3.5% per annum in accordance with NICE reference case.144
As CG146 recommends that either FRAX or QFracture is used to estimate the absolute risk of fracture,16 the simulation is run once using each of these tools to estimate fracture risk. First, it is run using QFracture to estimate the absolute risk of fracture. During this run the patient characteristics are stored. The model is then rerun using the same set of patients with identical characteristics but with the absolute risk of fracture being defined by FRAX rather than QFracture. This ensures that an identical patient cohort is simulated when using either QFracture or FRAX to estimate the absolute risk of fracture. In the deterministic model, random number control is used to ensure that the random numbers used are identical when running the same patient using both FRAX and QFracture. This eliminates the possibility that results achieved using the different risk calculators are different purely through chance. The same cohort of patients is run for each treatment and for each parameter sample during the PSA. This means that the 100th patient has the same characteristics and the same set of random numbers determining their path through the model regardless of the parameter samples selected for the PSA or the treatment being simulated.144 The DES model structure is represented in Figure 68.
Specifying the model population
The population included in the economic analysis is the whole population eligible for risk assessment within CG146. A heterogeneous population has been simulated and then stratified into risk categories based on absolute fracture risk, as predicted by either the FRAX or QFracture risk assessment tool. A heterogeneous population was simulated because we expected certain characteristics, such as age, which are not uniform across cohorts selected based on absolute risk, to have a non-linear relationship with cost-effectiveness. The population was stratified into risk categories to allow the variation in cost-effectiveness across absolute risk to be examined.
The NICE guideline on assessing the risk of fragility fracture (CG146)16 recommends that FRAX17 or QFracture18,19 should be used to assess the 10-year absolute risk of fragility fracture. Therefore, our analysis assumes that absolute fracture risk is measured using one of these two tools. (It is assumed that FRAX web version 3.9 and QFracture 2012 open-source revision 38 were used, as these were the versions available online at the time this report was prepared.) In both of these tools, absolute fracture risk is dependent on the patient’s age, sex, BMI and the presence or absence of a number of clinical risk factors. In the case of QFracture, ethnicity is also taken into account. In the case of FRAX, the patient’s BMD can also be incorporated if it is known, but CG146 recommends that BMD is measured only in patients whose absolute fracture risk falls close to a treatment threshold. Therefore, our model assumes that BMD is not known, as treatment thresholds must be defined for those without a BMD measurement for the recommendations in CG146 to be implemented. The FRAX tool estimates the individual’s 10-year absolute risk of hip fracture and their 10-year absolute risk of major osteoporotic fracture (clinical spine, hip, forearm and humerus fracture). The QFracture tool provides the absolute risk of hip and the absolute risk of major osteoporotic fracture (hip, spine, wrist or shoulder), but with the option to vary the timeframe from 1 year to 18 years (the web tool is limited to 10 years). Table 9 summarises the risk factors used by the FRAX and QFracture tools.
TABLE 9
Summary of risk factors included in FRAX (web v3.9) and QFracture (2012) tools
A particular level of absolute fracture risk, as measured by FRAX or QFracture, can be achieved in different ways by different individuals. For example, a young patient with many clinical risk factors may have the same absolute risk of fracture as an older patient who has no clinical risk factors. Although the absolute risk of fracture is likely to be an important determinant of the cost-effectiveness of treatment with bisphosphonates, other factors may affect cost-effectiveness independently of absolute fracture risk. For example, the cost and QALY consequences of fracture may be more severe in older patients, who may be more likely to die or be admitted to a nursing home following fracture. Therefore, in a group of patients who have been selected to have the same absolute fracture risk there may be variation in the cost-effectiveness of treatment. If there is a linear relationship between patient characteristics and cost-effectiveness, then it is possible to estimate the average cost-effectiveness by calculating the cost-effectiveness for a patient with average characteristics. However, previous work in this area suggests that cost-effectiveness may be non-linearly associated with patient characteristics, such as age.153 In such cases, an unbiased estimate of the mean cost-effectiveness can be achieved by simulating a patient population with heterogeneous patient characteristics and estimating the average cost-effectiveness across that population.154
In this analysis we have simulated a heterogeneous patient cohort that is representative of all patients eligible for risk factor assessment within CG146.16 We have limited the population to patients > 30 years, as neither the FRAX nor the QFracture tool has been validated in patients aged < 30 years. Initially, a population of patients aged ≥ 30 years is simulated, but only those eligible for risk factor assessment with CG146 are included within the cohort used within the cost-effectiveness analysis. For example, simulated patients without clinical risk factors (any included in QFracture or FRAX) are excluded from the analysis if they are female and aged < 65 years or male and aged < 75 years, and simulated patients are also excluded if they are aged < 50 years and do not have either a prior history of fragility fracture or current steroid use. This approach of sampling the whole population and then excluding those not recommended for risk factor assessment by CG146 was necessary, as data were not available on the distribution of clinical risk factors within the specific population eligible for risk assessment under CG146.
Once the cohort eligible for risk factor assessment was defined from within the general population, we estimated FRAX and QFracture scores for each individual (where ‘score’ refers to the absolute risk of fracture over 10 years for the four main fracture sites: hip, wrist, vertebra and proximal humerus). Lifetime costs and QALYs for each patient are then estimated using the cost-effectiveness model. This step is repeated once for no treatment and once for each bisphosphonate treatment strategy. We then stratified the patients into 10 risk categories based on their absolute fracture risk and estimated the average cost-effectiveness of each bisphosphonate compared with no treatment within each risk score category. The cut-off points for each risk category have been set using deciles to ensure that a sufficient number of patients fall into each category to allow the cost-effectiveness to be estimated accurately. The stratification into risk categories is done independently for QFracture and FRAX. As there is not necessarily agreement between the risk scores calculated by these two different risk assessment tools at the patient level, the same patients may not end up in the same risk category when using different tools to define absolute risks.
In order to stochastically sample patient characteristics we needed data on the prevalence of each clinical risk factor and the distribution of continuous factors, such as age and BMI. As well as considering the prevalence of individual risk factors, it is also important to determine whether or not there are correlations between any of the patient characteristics so that the sampling process can allow for the fact that some risk factors may be more likely to occur in the same patient than in separate patients. It is difficult to fully characterise the correlation structure of all of the risk factors that go into both the QFracture and FRAX tools without access to a database containing information on all of the risk factors in a large sample of patients. However, it is most important to capture the correlations between those characteristics that are likely to be significant determinants of cost-effectiveness independently of their impact on absolute fracture risk. This is because the prevalence of these factors will determine the distribution of cost-effectiveness within groups who have the same absolute fracture risk.
We developed a conceptual model outlining which risk factors are likely to significantly impact cost-effectiveness independently of their impact on absolute fracture risk. This was based on the relationships assumed in published models in this area, advice from our clinical advisors and rapid literature searches (Table 10). A summary of this conceptual model is shown in Figure 66. Age, sex, prior fracture, steroid use and residential status were identified as risk factors thought to affect cost-effectiveness independently of absolute fracture risk. Further details on the rationale for selecting these risk factors are given in Table 10. Ethnicity, family history of fracture and BMD were excluded, as these are expected to affect cost-effectiveness solely through their impact on absolute fracture risk. Although some of the remaining risk factors included in either FRAX or QFracture (e.g. alcohol use, smoking status, comorbidities, secondary causes of osteoporosis, medications, BMI and history of falls) might be expected to affect an individual’s baseline utility, life expectancy or likelihood of living in an institutional residential setting, these relationships were felt to be too weak to include within the model without adding unnecessary complexity to the model structure. Furthermore, many of these conditions are likely to be more prevalent within older patients or those living in residential care and, therefore, their impact on utility, all-cause mortality or outcomes following a fracture may already be captured by the relationship between these variables and age or residential status. We have therefore focused on trying to capture the correlations between age, sex, steroid use, prior fracture and residential status. This was achieved by looking for age- and sex-specific estimates of steroid use, prior fracture and residential status, as these were considered to be where the most significant correlations would lie. The conceptual model was developed to allow for the possibility that different efficacy data may be applied for different sexes and for steroid- and non-steroid-induced osteoporosis, but in the final analysis efficacy evidence was pooled across all included trials reporting fracture outcomes. The potential for increased all-cause mortality in steroid users was noted at the conceptual modelling stage, but no difference in life expectancy was applied in the final model.
TABLE 10
Patient characteristics expected to affect cost-effectiveness independently of absolute fracture risk
The primary data source used to characterise the patient population was the cohort used to derive the 2012 QFracture algorithm. This study used a large (n = 3,142,673) prospective cohort aged 30–100 years drawn from a large, validated primary care electronic database.18 This study was chosen as the primary source of data on patient characteristics as it was considered to be representative of the general UK population and provided data on all of the risk factors included within the QFracture algorithm. For the majority of the clinical risk factors, we used the prevalence within the 2012 QFracture cohort and applied the same prevalence across all ages and across both sexes. These risk factors are listed in Table 11 along with the prevalence reported for the 2012 QFracture cohort. Although many of these risk factors are expected to have varying prevalence across different sexes and age groups, it was not considered necessary to capture their correlation with age or sex, as they are assumed to influence cost-effectiveness through only their impact on absolute fracture risk.
TABLE 11
Clinical risk factors that were assumed to have a constant prevalence across the cohort
Although data were available on the age distribution for patients within the 2012 QFracture cohort, these data were not provided separately for males and females and the age profile of the UK population is known to differ slightly by sex.159 Therefore, sex-specific 2013 mid-year population estimates for England from the Office for National Statistics (ONS) were used to provide an empirical distribution for patient age.159 Figure 69 shows how the proportion falling within each band compares between the ONS data and the 2012 QFracture cohort. The data appear to be reasonably well matched except that the QFracture cohort appears to have a lower proportion in the 30–39 years category. The ONS data were considered to be more representative of the population in England and, therefore, the age of each individual patient was sampled using the sex-specific ONS data.

FIGURE 69
The proportion of those aged ≥ 30 years who fall within each age category. Based on ONS data and the age distribution in the 2012 QFracture cohort.,
The proportion of patients living in an institutional residential setting was estimated from the 2011 census data.160,161 Sex-specific data were available for 5-year age bands for all people who are usual residents in communal establishments.160 However, these 5-year estimates included people resident in other types of communal establishments such as children’s homes and prisons. Data were also available on specific types of establishments for 10-year age bands.161 We selected data for people resident in medical and care establishments, which included NHS, local authority and other establishments both with and without nursing care. We then used the 5-year data on all communal establishments to divide up the 10-year data into 5-year age bands. These data, shown in Figure 70, were used to sample whether or not an individual was living in an institution according to their age and sex.
For steroid use, data published by van Staa et al.162 suggest that the prevalence of current steroid use increases with age. Their estimates were based on analysis of the GPRD (which is now called Clinical Practice Research Datalink), which is a large database of GP records for UK patients. This provided a large retrospective cohort that is likely to be representative of the general population of England and Wales. Data on the prevalence of oral glucocorticoid use by sex and 10-year age bands were digitally extracted from a graph provided by van Staa et al.162 The relationship between prevalence and age appear to follow a similar pattern for low-, medium- and high-dose users. Data were extracted for only medium-dose (2.5–7.5 mg per day) and high-dose (≥ 7.5 mg per day) steroid users as these dosages overlapped with the range specified in the FRAX fracture risk algorithm (≥ 5 mg per day). However, when these data were combined with the ONS data on the current age distribution within England to estimate the average prevalence across patients aged ≥ 30 years, this was substantially lower than the prevalence recorded in the QFracture database (0.95% vs. 2.2%). The difference may be because we did not include low-dose users from the van Staa et al.162 estimates or that the QFracture data do not appear to relate to a specific dose of steroids. A more recent estimate of the prevalence based on UK GP records is provided by Fardet et al.163 Although this did not provide a breakdown of the prevalence by age and sex, the overall prevalence of 0.79% for 2008 reported by Fardet et al.163 is closer to that reported by van Staa et al.162 than the figure reported in the QFracture database. We therefore decided to use the combined data for medium- and high-dose users provided by van Staa et al.’s data to characterise the age and sex distribution of steroid use. Figure 71 shows age- and sex-specific prevalence estimates applied in the model for steroid use.

FIGURE 71
Prevalence of current steroid use: data from van Staa et al. combined for medium- and high-dose steroid users.
Data on the prevalence of previous fracture were taken from a meta-analysis by Kanis et al.164 This study was selected as it provided data on the prevalence of prior fracture, reported by sex and 10-year age bands. The cohorts used to estimate the prevalence of prior fracture were the same cohorts used to estimate the impact of prior fracture on future fracture risk for the FRAX algorithm.17 The prevalence of prior fracture is difficult to quantify as it depends on whether or not all prior fractures are included regardless of the site of fracture or the mechanism of injury. Although the definitions used varied across the multiple cohorts that informed the estimates from Kanis et al.,164 the fact that these cohorts were then used to derive the impact of prior fracture on future fracture risk provides some consistency between the definition of prior fracture used for prevalence and for risk score calculation. The prevalence reported by Kanis et al.164 for each of the 10-year age bands, which ranged from 15% at age 30 years to 48% at age 80 years in women, is much higher than that reported within the QFracture cohort (1.9% across a cohort aged ≥ 30 years).18,164 An alternative estimate of the prevalence of prior fracture is provided by Scholes et al.,165 who used data collected during the Health Survey for England to estimate the prevalence of previous fracture in community-dwelling people aged > 55 years. They found that the prevalence was 49% in men and 40% in women, although these data relied on the individuals’ recall and did not distinguish between fragility fractures and those occurring in early life or associated with significant trauma. Another source of evidence that can be used to cross-check the estimates provided by Kanis et al.164 are studies reporting the incidence of fracture by age. Prevalence can be estimated from these studies in an approximate manner by assuming that the prevalence of prior fracture at a particular age is equivalent to the cumulative incidence across all previous age bands although, under this assumption, the prevalence may be inflated by multiple fractures occurring within the same patient, if these are reported separately in the incidence data.
Data on the incidence of fracture by age and sex and the proportion of fractures that are fall related (standing fall, fall down stairs or fall from a low height) are provided by Court-Brown et al.166 This was a prospective cohort study conducted in Scotland in 2010/11 that compared the rate of fractures presenting to the Royal Infirmary of Edinburgh with population estimates from the 2001 census to estimate incidence rates. Estimating the prevalence of fall-related fractures from these data, by assuming that it is equal to the cumulative incidence in those aged over 35 years, provides prevalence data closer to those reported by Kanis et al.164 than those reported in the QFracture cohort. Therefore, the data presented by Kanis et al.164 (Figure 72) were used in the model to sample the likelihood of an individual having a prior fracture.164 A second incidence study, by van Staa et al.,167 provides data on the incidence of fracture in England in a general practice (GPRD) cohort, which examined over 20 million person-years of follow-up. The proportion of fractures that were fall related in the study by Court-Brown et al.166 was applied to the incidence data reported by van Staa et al.167 to estimate the incidence of fall-related fractures in an attempt to exclude fractures related to significant trauma such as road traffic accidents. Prevalence of a prior fracture after the age of 3 years was then estimated by calculating the cumulative incidence from age 20 years, and these data are summarised in Figure 73. The prevalence estimated in younger age groups, when using this method, was lower compared with the data reported by Kanis et al.164 This alternative estimate of the prevalence of prior fracture was applied in a sensitivity analysis to assess whether or not the cost-effectiveness of bisphosphonate treatment is sensitive to the prevalence of prior fracture in the population.

FIGURE 72
Proportion who have had a prior fracture by sex and age band (data applied in base case). From Kanis et al.

FIGURE 73
Proportion who have had a prior fracture by sex and age band (data applied in sensitivity analysis). Figures presented were calculated by adjusted data from van Staa et al., using additional data from Court-Brown et al.
Swedish estimates for the incidence of fracture at different sites across sexes and age bands were then used to estimate the cumulative prevalence of fractures at various sites up to the start age for each age band.148 These data were used to determine the distribution of prevalent fractures across different fracture sites, as shown in Table 12. As the incidence data were presented for patients aged ≥ 50 years we have assumed that the distribution of prior fractures at ages 30–55 years is equal to the distribution of incidence of fracture from ages 50–55 years. It can be seen that as the incidence of hip fracture rises with age, the proportion of prior fractures that have occurred at the hip increases with each increasing age category.
TABLE 12
Distribution (%) of prevalent fractures across the four main osteoporotic fracture sites (within each sex)
Data are available from the Health Survey for England on the average BMI for different ages and sexes.168 These data, presented in Figure 74, show that BMI varies with age. Although BMI is not expected to affect cost-effectiveness except through its influence on absolute fracture risk, it is considered to be an important risk factor, particularly where BMD is unknown. A 2014 meta-analysis found that the relationship between BMI and fracture risk is much weaker after adjusting for BMD.169 A significant positive correlation was also found in this study between BMI and BMD (95% CI 0.32 to 0.33, r = 0.33; p < 0.001). Given the significant correlation between these two variables and the fact that we are assuming that BMD is not available when fracture risk is first assessed, we decided to model the age variation in BMI, as this may capture some of the underlying variation in BMD with age. However, we accept this will capture only a small proportion of the association between BMD and age. We decided to use the Health Survey for England data168 to characterise the mean BMI for different age bands and sexes, as these data allow the SD to be calculated. However, they do not provide any information on the shape of the BMI distribution. We assumed that the BMI values were log-normally distributed, as we found that assuming a normal distribution overestimated the proportion falling within the underweight category. As it is the underweight group who are at particular risk of a fragility fracture, assuming a normal distribution would have overestimated population fracture risk.169 As can be seen in Figure 75, assuming a log-normal distribution still overestimated the proportion who were underweight, but by a factor of 3 rather than 5.

FIGURE 74
Mean BMI by age and sex from 2012 Health Survey for England.

FIGURE 75
Proportion of men (adults aged ≥ 16 years) falling into different weight categories. HSE, Health Survey for England.
Treatment strategies
The model compares the following treatment strategies:
- alendronic acid (10 mg per day or 70 mg per week)
- risedronic acid (5 mg per day or 35 mg per week)
- ibandronic acid (150 mg per month)
- i.v. ibandronic acid (3 mg every 3 months)
- zoledronic acid (5 mg/year)
- no treatment.
We have not distinguished in the model between the daily and weekly formulations of alendronic acid and risedronic acid, as the weekly formulations for these are considered to be clinically equivalent and the effectiveness evidence has not been analysed separately for weekly and daily doses.
We assume that all patients will receive adequate supplemental calcium and vitamin D regardless of whether or not they are being treated with a bisphosphonate and, therefore, no cost is included within the model for calcium and vitamin D supplements. Patients in the no-treatment arm are assumed to receive no further treatment to reduce their fracture risk. We have not assumed any active follow-up for patients receiving either bisphosphonates or no treatment.
We assume that the intended treatment duration is 5 years for alendronic acid, risedronic acid and ibandronic acid (both oral and i.v.) and 3 years for zoledronic acid. However, not all patients persist with therapy for the intended duration, as previously discussed in Chapter 3, which describes the clinical evidence on treatment persistence. The duration of treatment in the model was therefore set to the mean duration of persistence using data from the systematic reviews described in Chapter 3. The highest-quality systematic review was considered to be that by Imaz et al.,128 which reported that the mean duration of treatment persistence was 184 days (95% CI 164 to 204 days) for oral bisphosphonates (alendronic acid, risedronic acid and ibandronic acid). Only one of the studies included in the meta-analysis of average persistence by Imaz et al.128 examined ibandronic acid, with the rest considering alendronic acid and risedronic acid. However, the mean duration of persistence for monthly ibandronic acid was similar to the mean duration for weekly alendronic acid and risedronic acid (98 days for ibandronic acid vs. 116 days and 113 days for alendronic acid and risedronic acid, respectively). Therefore, we decided to use the pooled estimate provided by Imaz et al.128 for all oral bisphosphonates.
The review by Imaz et al.128 did not provide any data on persistence in patients receiving i.v. bisphosphonate therapy.128 However, a review by Vieira et al.132 identified a cohort study (Curtis et al.170) in US Medicare patients that provided estimates of the mean number of infusions received for zoledronic acid and i.v. ibandronic acid.170 It is noted that the duration of treatment with zoledronic acid estimated by Curtis et al.170 was considered by our clinical advisors to be low compared with their own experience of administering zoledronic acid within clinical practice. However, in the absence of an alternative estimate these data were used to estimate the mean duration of persistence with therapy for i.v. bisphosphonates. The full treatment effect was assumed to persist for 1 year after the last zoledronic acid infusion and 3 months after the last ibandronic acid infusion. Persistence data applied in the base-case model are summarised in Table 13. A sensitivity analysis in which we assumed full persistence with treatment for 3 years for zoledronic acid and 5 years for all other treatments was also examined.
TABLE 13
Duration of persistence with treatment
The fall-off period was assumed to be equal to the duration of treatment for all treatments except zoledronic acid, for which a longer fall-off period was assumed. Clinical advice was that a 7-year fall-off period could be assumed for 3 years of zoledronic acid treatment. We therefore assumed an approximate fall-off period of 2.33 (= 7/3) times the treatment period for zoledronic acid.
Estimating time to event from absolute fracture risk
Time to fracture has been estimated by fitting a parametric survival function to the estimates of absolute risk provided by the QFracture algorithm. For the model using FRAX, the parametric form and shape parameter fitted to the QFracture data has been used but the rate parameter of the survival function has been adjusted to ensure that the absolute fracture risk at 10 years, predicted by the survival function, matches that predicted by the FRAX tool. Treatment effects are incorporated by applying a HR to the rate parameter, with further details on the incorporation of treatment efficacy provided in Incorporating the risk of fracture at other sites and Application of hazard ratios to incorporate treatment effects both during and beyond the treatment period.
The algorithm used by the QFracture tool to calculate the risk of fracture over varying time periods is publicly available on the QFracture website (www.qfracture.org/). This algorithm was examined and was found to have the following form:
where the parameter η is the risk-modifying factor that adjusts for patient characteristics and S0 is the underlying survival function. Different values of S0 are defined according to the time frame (t) over which risk is to be assessed. The survival model used to estimate the risk-modifying factor η is described as a Cox regression. In a Cox regression the values for S0 do not have to follow any particular parametric form. However, when the S0 values were plotted, to give the fracture-free survival for patients without any risk-modifying factors (η = 0), it was noted that they appeared to be very smooth, suggesting that it may be possible to fit a functional form to the underlying survival function. Given that the Weibull function (which includes the exponential function as a special case) and the Gompertz function are both compatible with proportional hazards assumptions, we tested both of these parametric forms to see if they were suitable.
A plot of ln(–ln(S(t))) against ln(t) was produced to see whether or not the data were consistent with a Weibull survival curve. This was done for an example patient with the following characteristics: female, aged 50 years, BMI of 24 kg/m2 and no clinical risk factors. The same plot was then produced for a patient with type 1 diabetes but no other clinical risk factors and the same age and BMI to examine the impact of clinical risk factors on the shape of the plots. From Figure 76 it can be seen that the distance between the plots is constant for these two cases, as would be expected for a proportional hazards model, but neither plot is linear over the whole time period. The plots appear to be linear over short time periods (5 or perhaps 10 years), but the Weibull curve does not appear to be appropriate over longer time frames.

FIGURE 76
Plot to test suitability of Weibull survival curve. Patient characteristics: female, aged 50 years, BMI of 24 kg/m2, with or without type 2 diabetes.
A plot of ln(hazard) against time was generated once again for a 50-year-old female with a BMI of 24 kg/m2 and either with or without type 2 diabetes as shown in Figure 77. This was found to be linear, suggesting that the underlying survival function was consistent with a Gompertz distribution. We have therefore assumed that the underlying survival function follows a Gompertz distribution and used the linear fit for the ln(hazard) function to estimate the parameters for the Gompertz distribution in patients without any risk-modifying factors (η = 0). Table 14 shows the survival parameters for the underlying Gompertz distribution in males and females for the outcomes of hip fracture-free survival and osteoporotic fracture-free survival with osteoporotic fracture defined as hip, wrist, vertebral or proximal humerus fracture.

FIGURE 77
Plot to test suitability of Gompertz parametric form. Patient characteristics: female, aged 50 years, BMI of 24 kg/m2, with or without type 2 diabetes.
TABLE 14
Parameters for fitted Gompertz functions in patients with no risk-modifying factors (η = 0)
Figures 78–81 show the fit of the parametric curve against the survival data specified in the QFracture algorithm for each of these survival functions. It can be seen from the plots that the parametric curves fit the data better in the first 10 years and that the parametric curves may underestimate long-term fracture risk. Although this was noted as a limitation, the good fit up to 10 years means that the rates are sufficiently accurate during the period in which drugs are assumed to affect fracture outcomes. An underestimation of the long-term fracture risk in the period after the drug efficacy is assumed to fall to zero is likely to affect all treatment strategies equally and, therefore, is not expected to significantly bias the estimates of cost-effectiveness. We therefore assumed that the fitted Gompertz curve could be used to estimate time to fracture for patients with no risk-modifying factors.

FIGURE 78
Gompertz fit for a female patient with no risk-modifying factors (η = 0) for the outcome of any osteoporotic fracture (hip, wrist, proximal humerus or vertebral).

FIGURE 81
Gompertz fit for a male patient with no risk-modifying factors (η = 0) for the outcome of hip fracture.

FIGURE 79
Gompertz fit for a female patient with no risk-modifying factors (η = 0) for the outcome of hip fracture.

FIGURE 80
Gompertz fit for a male patient with no risk-modifying factors (η = 0) for the outcome of any osteoporotic fracture (hip, wrist, proximal humerus or vertebral).
QFracture does not provide individual predictions for each of the four major osteoporotic fractures (hip, wrist, vertebral and proximal humerus). Instead, it provides an estimate of the absolute risk of fracture across all four fracture types. In order to provide an estimate of the time to fracture for each site, we multiplied the alpha parameter for the fitted Gompertz survival curve by the proportion of patients experiencing an incident fracture of that type. The proportions, shown in Table 15, were estimated from Kanis et al.148 and provide the incidence of fractures in Sweden across different fracture sites by sex and age band.
TABLE 15
Proportion (%) of major osteoporotic fractures occurring at each site by sex and age band
We used these site-specific alpha values to generate samples from the Gompertz distribution for each fracture site and plotted a survival function for time to fracture at each site. To validate this approach, of apportioning the alpha value for major osteoporotic fracture across the four sites, we calculated the time to first major osteoporotic fracture from these site-specific fracture survival curves and compared these to the survival from major osteoporotic fracture predicted by the QFracture algorithm. We found that the survival curves generated were comparable, suggesting that this method of calculating site-specific fracture curves is valid, as can be seen from Figure 82.

FIGURE 82
Plot of survival curves for time to fracture based on 10,000 patients for each individual fracture site and for any major osteoporotic fracture.
However, as can been seen from Figure 83, when we compared the hip fracture data calculated from major osteoporotic fracture to the hip fracture survival estimates provided directly from the QFracture algorithm, we found that these did not match well over longer time frames (i.e. over 5 years). This can be explained by the fact that the beta value for the hip fracture-specific Gompertz curve is higher, suggesting a faster increase over time for hip fracture than is seen over all major osteoporotic fractures. We decided to use the hip fracture survival predicted by apportioning the major osteoporotic fractures in the base-case analysis, as this would provide an estimate of major osteoporotic fracture that is consistent with the estimates from the QFracture algorithm. Furthermore, the beta value for the Gompertz function for major osteoporotic fracture is likely, in reality, to be the average of a lower value for non-hip and a higher value for hip, but as the non-hip value could not be calculated we felt it was better to use the beta value for major osteoporotic fracture and apply it to all four fracture types in the base-case analysis. A sensitivity analysis was also conducted using the hip-specific algorithm from QFracture for estimating time to hip fracture to see whether or not this had a significant impact on the cost-effectiveness.

FIGURE 83
Comparison of survival curves from sampling directly from the Gompertz for hip fracture and from sampling hip as a proportion of the Gompertz curve for major osteoporotic fracture against the source QFracture data for hip.
The following method was used to calculate time to event for each fracture type in the base-case analysis when assuming that patients have been assessed using the QFracture algorithm.
- calculate the proportion, p, of major osteoporotic fractures that occur at the site of interest according to the person’s age and sex
- calculate the risk score modifier, η, from the patient characteristics
- select the beta for the sex-specific Gompertz survival curve
- select HR, which incorporates any treatment effect from intervention
- calculate alpha for the sex-specific Gompertz survival curve as follows:
- sample time to fracture from Gompertz (alpha, beta)
A similar approach was not possible when estimating time to event using the estimates of absolute fracture risk provided by the FRAX algorithm. This is because the algorithm used to calculate absolute fracture risk within the FRAX tool is not publicly available and, therefore, it was not possible to assess whether or not survival from fracture follows a particular parametric form. Instead, we assumed the underlying shape of the survival curve for FRAX would be identical to that used in the QFracture algorithm. In effect this meant assuming a Gompertz curve is followed, which has the same beta parameter as seen in the QFracture algorithm. In doing so, we were then able to calculate the time to event for patients assessed using the FRAX tool by calculating the multiplier, Φ, which needed to be applied to the alpha value of the QFracture survival curve to provide the absolute risk of fracture at 10 years, predicted by FRAX. In doing so, we assumed that there is a constant HR between the number of events predicted by FRAX and the number predicted by QFracture across all time frames. From Equations 2–4, it can be seen that Φ can be calculated by comparing the absolute risk of fracture estimated by the two fracture risk tools.
Absolute risk at 10 years in FRAX:
Absolute risk at 10 years in QFracture:
From this we can derive that:
One of the complicating factors with this approach is that QFracture provides an estimate of fracture risk without the competing risk of mortality, whereas FRAX provides an estimate of absolute fracture risk when taking into account the competing risk of mortality. Therefore, at older ages, when the risk of mortality is higher, the FRAX algorithm will calculate lower estimates of 10-year risk than the QFracture algorithm. It was not possible to correct for this within our model, as we did not have sufficient information regarding the competing hazard of death used within the FRAX algorithm to adjust the FRAX estimates to exclude the competing risk of mortality.
Incorporating the risk of fracture at other sites
Fractures at additional sites (femoral shaft, humeral shaft, pelvis, scapula, clavicle, sternum, ribs, tibia and fibula) have been incorporated by increasing the incidence of fractures at the four main sites (hip, wrist, spine and proximal humerus). Although several of the published cost-effectiveness analyses restricted the fracture types to the four main sites,137,139,140 some of the studies incorporated fractures at additional sites45,49–106,108–143 by grouping these with one of the four main fracture sites. The decision over which fractures to group together has, in previous analyses, been justified by the expectation of similar costs and disutilities across particular groups of fractures.171 The groupings used were consistent across the three published cost-effectiveness analyses that incorporated additional sites.141–143
We decided to keep the groupings used in these three studies with one exception. These studies grouped pelvic fractures with hip fractures. Pelvic fractures associated with osteoporosis were considered by our clinical advisors not to be associated with an excess risk of mortality similar to that associated with hip fractures and the costs were also expected to be lower. Therefore, pelvic fractures were grouped instead with proximal humerus fractures. Therefore, the grouping of fracture sites used within our model was as follows:
- femoral shaft grouped with hip
- clavicle, scapula, rib and sternum grouped with wrist
- tibia, fibula, pelvis and humeral shaft grouped with proximal humerus.
Both QFracture and FRAX use a clinical definition for vertebral fractures and, therefore, the rate of vertebral fractures predicted in our model is specific to clinical vertebral fractures. The cost and quality-of-life implications of morphometric vertebral fractures that are not clinically apparent are likely to be much smaller than for clinically apparent vertebral fractures. Therefore, we expect that excluding morphometric fractures that are not clinically apparent from the model to have a small impact on the ICER. Previous analyses by Stevenson et al.140 (reported in appendix 15 of their monograph) suggest that the exclusion of morphometric fractures does not significantly bias the estimates of cost-effectiveness.
The multipliers applied to the rate of hip, wrist and proximal humerus fractures to incorporate the additional fractures sites were calculated based on Swedish incidence data reported by Kanis et al.148 and are shown in Table 16. These were applied in the model to the alpha parameter for the Gompertz sampling of time to fracture. As the alpha parameter is the rate parameter for the Gompertz survival curve, a multiplier > 1 increases the risk of fracture. The data from age band 50–54 years were applied to those aged 30–50 years. The very high multiplier for wrist fractures in men is driven by a large incidence of rib fractures compared with wrist fractures in the data reported by Kanis et al.148
TABLE 16
Multipliers applied to the rate of hip, wrist and proximal humerus fractures to include fractures at other sites (calculated from incidence data reported by Kanis et al.)
Application of hazard ratios to incorporate treatment effects both during and beyond the treatment period
As we have assumed a Gompertz underlying survival function for time to fracture, and as this is a proportional hazards model, the HR for treatment can be applied directly to the alpha parameter as described in Estimating time to event from absolute fracture risk. When taking a proportional hazards approach the treatment effect, as measured by the HR, is assumed to be constant over the entire duration of the survival curve. However, bisphosphonates are commonly given for only a few years and, therefore, we needed the model to allow for a fall-off in treatment effect after treatment is finished. For patients who complete the intended treatment period (5 years for all bisphosphonates except zoledronic acid) we have assumed a linear fall-off in HR for each year from years 5 to 10 such that the HR at 10 years is 1. For zoledronic acid, we have assumed a 3-year treatment period and a linear fall-off in treatment effect from years 3 to 10 such that the HR is 1 at year 10. This has been done by resampling the time to fracture at the end of the treatment period and applying a HR modified to account for the fall-off in treatment from years 5 to 10. The HR is modified by taking the average HR for full treatment effect and zero treatment effect. This modified HR is applied for the duration of the fall-off period. Although this linear approximation may underestimate the treatment effect in the early years after stopping and overestimate it in the later years, it should provide the correct treatment effect on average over the fall-off period. Adding more dummy events to update the HRs at more frequent intervals over the fall-off period was avoided, as it would reduce the computational efficiency of the model.
The time to fracture is resampled at the end of the fall-off period, with a HR of 1 applied thereafter. As the HR is assumed to increase over time in a Gompertz survival curve, the patient’s age is updated prior to resampling the time to fracture resulting in a new alpha value in the Gompertz function. We noted that the QFracture algorithm does not appear to be internally consistent when applied at different ages. For example, the 1-year risk of fracture in a 55-year-old is lower than the 1-year risk of fracture predicted for the fifth year in a patient aged 50 years. Given this internal inconsistency within the QFracture algorithm, our method of resampling at 5 and 10 years results in a stepped linear function for the ln(hazard) even when the HR is held constant over the whole modelled timeframe. However, this method maintains the proportional hazards assumption within each step. This can be seen in Figure 84, where the diamonds and squares show the stepped ln(hazard) function which results from resampling at 5 and 10 years when applying a constant HR of 2 or 1, respectively. It can be seen that the gap between the diamonds and squares is constant across the whole timeframe as would be expected for a proportional hazards model. Figure 85 demonstrates the additional effect of modifying the HR at 5 and 10 years to allow for reduced treatment effect during the fall-off period and no treatment effect beyond the fall-off period. It can be seen that this brings the ln(hazard) function for the treated patients (with treatment associated with a HR of 2 in this example), shown by the squares, down to match that of the no-treatment group (constant HR = 1 across all years), shown by the diamonds, from 10 years as would be expected. It should be noted that the squares and diamonds in Figure 85 do not match exactly, as the graphs are based on stochastic time-to-event estimates but we would expect them to match exactly if an infinite number of samples were used to derive the plotted points.

FIGURE 84
Plot showing how resampling at 5 and 10 years results in a stepped ln(hazard) plot, but maintains the gap associated with the HRs.

FIGURE 85
Plot showing the effect of adjusting the HRs to reflect falling treatment effect during the fall-off period (5–10 years) and after the fall-off period (> 10 years).
In those scenarios where we assume that patients do not persist with treatment for the full 5 years (or 3 years for zoledronic acid), we have used additional dummy events at 5 and 10 years to ensure that all patients receive an updated estimate of fracture risks at these time points.
Efficacy estimates
The HRs for fracture estimated by the systematic review and NMA described in Chapter 3 have been applied in the model. Fracture data have been synthesised using an NMA model including all studies defined by the inclusion/exclusion criteria (i.e. males and females, steroid users and non-steroid users, confirmed low BMD or BMD unknown). The resulting measure of treatment effect was a HR for the effect of each bisphosphonate relative to placebo together with an estimate of the between-study SD.
The NMA described in Chapter 3, Methods for the network meta-analyses, has been used to generate the joint predictive distribution of the HR for each treatment compared with no treatment in a new study; this acknowledges heterogeneity in the effect of each treatment depending on the characteristics of patients included in the studies. These relative treatment effects have been applied consistently across the whole modelled population within the economic analysis.
Absolute effects of treatment predicted by the economic model (e.g. number needed to treat) vary across the population because of some patients having a higher absolute risk of fracture based on either their QFracture or FRAX score.
The effect of treatment on hip fracture was estimated from studies reporting hip fracture data. The effect of treatment on vertebral fractures was estimated from studies reporting all vertebral fractures (i.e. clinical and morphometric) because not all studies (i.e. treatments) reported outcomes for clinical vertebral fracture. The effect of treatment on proximal humerus fractures was estimated using all non-vertebral fractures as a proxy because too few studies reported data for fractures specifically at the proximal humerus. Evidence on the effect of treatment on wrist fractures was available for all treatments except for zoledronic acid. The effect of zoledronic acid was estimated from the statistical model using the predictive distribution of a new bisphosphonate in a population of bisphosphonates.
The efficacy evidence from 2.5 mg per day of oral ibandronic acid has been applied to both 150 mg per month of oral ibandronic acid and 3 mg per 3 months of i.v. ibandronic acid where no alternative fracture data were available for these licensed regimens, as the monthly oral and quarterly i.v. doses were licensed based on their non-inferiority in lumbar spine BMD outcomes when compared with the daily ibandronic acid treatment regimen.45,47,49,172,173 Where there were fracture data available for monthly oral ibandronic acid but none for quarterly i.v. ibandronic acid or daily oral ibandronic acid, we have assumed that the data from the monthly oral treatment can be applied to the i.v. treatment regimen. This was considered to be reasonable, as both the oral monthly dose and the quarterly i.v. dose were licensed based on non-inferiority compared with the daily oral dose for lumbar spine BMD outcomes.172,173 Our own analysis of the femoral neck BMD data for these treatments would support this assumption of similar treatment effects for oral monthly ibandronic acid and quarterly i.v. ibandronic acid.
Fractures occurring at sites other than one of the four main osteoporotic fracture sites have the efficacy applied according to the site groupings previously described, that is hip fracture efficacy data are applied to other femoral fractures; wrist fracture efficacy data are applied to scapula, clavicle, rib, sternum; and all non-vertebral fracture efficacy data are applied to tibia and fibula, pelvis and humeral shaft.
The HR is assumed to be constant over the duration of the treatment period and then to decrease linearly over the fall-off period, reaching no effect by the end of the fall-off period. The linear fall-off is approximated by applying the average HR of full and zero treatment effect for the duration of the fall-off period.
The HRs applied in the base case are shown in Table 17. The median HRs estimated by the NMA were used in the deterministic analysis, and in the PSA the convergence diagnostics and output analysis samples from the NMA were used as these preserve the underlying joint distribution.
TABLE 17
Hazard ratios applied in the deterministic analysis
Adverse event estimates
The model incorporates one-off costs and QALY decrements associated with AEs experienced in the first month of treatment. The published economic evaluations described in Chapter 4, Systematic review of existing cost-effectiveness evidence, were examined to see how they incorporated AEs. For oral bisphosphonates, the approach taken was based on the approach used by Stevenson et al.,140 who incorporated AEs for upper GI symptoms in their analysis. For i.v. bisphosphonates the rates of flu-like symptoms were taken from the RCT evidence summarised in Chapter 3 and the quality-of-life decrement was based on an ad hoc search. The data used to incorporate AEs within the model are described in detail below and summarised in Table 18.
TABLE 18
Summary of AE data applied in the model
We have not explicitly modelled the relationship between AEs and treatment persistence, but it is expected that AEs contribute to the low levels of treatment persistence described in Treatment strategies.
Adverse events associated with bisphosphonate treatment were not consistently incorporated in economic analyses included in our review. Stevenson et al.140 did not include any AEs in the model reported in their 2005 publication, but a later Decision Support Unit (DSU) report by Stevenson and Davis174 describes additional analyses in which AEs were included. Both Kanis et al.138 and Borgström et al.137 used the assumptions described in the DSU report by Stevenson et al.174 within sensitivity analyses, but neither included AEs in their base case. The remaining published analyses139,141–143,146 did not include AEs.
Stevenson et al.174 used data from prescription event monitoring studies identified in a systematic review by Lloyd-Jones and Wilkinson116 to determine the rate of upper GI problems in patients treated with oral bisphosphonates. In the DSU report, Stevenson et al.174 assumed 2.35% of patients required a GP appointment and a course of H2 receptor antagonists as a result of GI AEs in the first month of therapy and 0.35% thereafter.174 These patients were assumed to have a HRQoL decrement of 9% (utility multiplier of 0.91 from Groeneveld et al.175) for the full month, which was described by Stevenson et al.174 as a deliberately pessimistic assumption that aimed to counterbalance the fact that no other adverse AEs, such as nausea, had been included. Lloyd-Jones and Wilkinson116 also reported that other cohort studies found that 30% of patients starting alendronic acid may report GI AEs. A sensitivity analysis using a higher rate of AEs (24%) in the first month of alendronic acid treatment was considered by the Technology Appraisal Committee when formulating recommendations for TA16021 and TA161.24
Our review of systematic reviews examining AEs did not identify any systematic reviews that examined GI AEs that were published more recently than the review by Lloyd-Jones and Wilkinson.116 The prescription event monitoring studies identified by Lloyd-Jones and Wilkinson116 found a greater incidence of dyspeptic conditions in the first month of treatment with alendronic acid and risedronic acid (3%) than in later months (1%).116 This was considered by our clinical advisors to be low compared with the rates they saw in clinical experience, which were estimated to be around 20%.
All three oral bisphosphonates were found to have similar rates of GI symptoms when compared with placebo in RCTs. Furthermore, prescription event monitoring data and data from two head-to-head RCTs suggest similar rates of GI symptoms for alendronic acid and risedronic acid. The consultee submission by Actavis176 cited a study by Ralston et al.,126 which concluded that switching patients who are stabilised on risedronic acid to alendronic acid is associated with an increased risk of GI AEs. However, this evidence was not considered to be directly applicable to the question of whether or not AEs are more common when initiating treatment with alendronic acid or risedronic acid in patients without prior treatment with bisphosphonate. Limited data were available to assess if monthly formulations result in a lower incidence of GI symptoms than weekly formulations, but the review by Bobba et al.112 stated that increasing the dosing interval to weekly or monthly does not appear to change the rates of GI AEs when compared with daily dosing for any of the three oral bisphosphonates. Therefore, the rates of AEs for alendronic acid from prescription event monitoring studies have been applied consistently to all oral bisphosphonates. Our clinical advisors informed us that clinical experience would suggest that upper GI symptoms are most problematic for alendronic acid, with risedronic acid being less problematic and ibandronic acid even less so owing to less frequent dosing. However, as this evidence was anecdotal, they considered it reasonable to assume equivalent AEs for the oral bisphosphonates.
In the model we applied the data on dyspeptic conditions from prescription event monitoring studies described by Lloyd-Jones and Wilkinson116 and assumed that 3% of patients starting treatment with an oral bisphosphonate experience GI symptoms requiring a GP appointment and prescription of a H2 receptor antagonist in the first month of treatment. A sensitivity analysis was also conducted examining a rate of 30% in the first month to reflect the higher rates observed in some observational studies, as described by Lloyd-Jones and Wilkinson.116 Clinical advice was that proton pump inhibitors are usually prescribed instead of H2 receptor antagonists despite a caution in the British National Formulary regarding the potential for an increased fracture risk for proton pump inhibitors.39 However, as generic lansoprazole (Zoton FasTab®, Pfizer) is similarly priced to generic ranitidine, we have assumed for simplicity that all patients receive a H2 receptor antagonist. Total cost per patient experiencing a GI AE was assumed to be £46.76 (£45 for GP appointment and £1.76 for generic ranitidine).39 We have applied the same assumptions on disutility as Stevenson et al.,140 which we calculate to be equivalent to a QALY loss of 0.0075 per patient experiencing GI symptoms. We have applied this as a fixed QALY decrement at the start of the model without adjustment for baseline health utility.
In our review of AEs, the incidence of flu-like symptoms was found to be significantly higher among patients treated with zoledronic acid than among those receiving placebo. Although none of the RCTs or observational studies reported flu-like symptoms for i.v. ibandronic acid, the SmPC for Bonronat® (Roche Products Ltd) (branded i.v. ibandronic acid) describes influenza-like symptoms that resolve after ‘a couple of hours/days’ as a common side effect, affecting up to 1 in 10 people. A study by van Hoek et al.177 reports the utility for influenza-like illnesses as being 0.34 compared with a baseline (no flu-like symptoms) of 0.97 based on EQ-5D scores in a cohort of 655 patients with influenza-like illness. Based on these estimates, we considered that a utility multiplier of 0.35 would be reasonable for flu-like symptoms. We have assumed a disutility of 0.65 for 3 days for flu-like symptoms associated with i.v. bisphosphonates, which is equivalent to a QALY loss of 0.005. This has been applied as a fixed QALY decrement at the start of the model without adjustment for baseline utility. We took the rate of influenza-like symptoms to be the rate of pyrexia reported in the HORIZON-PFT,56 as this was the largest RCT reporting data on flu-like symptoms and pyrexia was more common than other flu-like symptoms (headache/chills). The 14% difference in pyrexia rates between zoledronic acid and placebo was applied to patients receiving either i.v. zoledronic acid or i.v. ibandronic acid. These were applied for only the first infusion to reflect the fact that these rates were measured over the whole trial period (36 months) and, therefore, applying them repeatedly would overestimate the incidence of flu-like symptoms. Furthermore, it is likely that patients who experience significant side effects are more likely to be in the group who do not persist with treatment so repeated episodes of significant disutility are unlikely.
Estimating time to non-fracture-related mortality
Sex-specific UK life tables were used to provide an empirical estimate of the likelihood of death for each year after the start of the model.155 This was calculated based on the age of the patient. So for a patient aged 30 years, the likelihood of death (denoted by dx within the life tables) between each birthday from the age of 30 to100 years was used to estimate the empirical distribution of survival times. Similarly, for a patient aged 90 years, the likelihood of death between each birthday from age 90 to 100 years was used. This method assumes no survival beyond age 100 years, as this is the limit of the data provided in the life tables. The time horizon of the model was therefore set to equal 100 years minus the starting age, giving a variable duration modelled depending on the patient’s start age. The data used to estimate time to non-fracture-related death were not varied in the PSA.
Mortality after hip fracture
Patients experiencing hip fracture in the model have a risk of experiencing fracture-related mortality, which is applied as a one-off probability at the time of fracture. The risk is age and sex specific, and fracture-related death is assumed to occur at a fixed time following fracture rather than at a sample time in the future. All of the published cost-effectiveness analyses described in Systematic review of existing cost-effectiveness evidence included an excess of risk of mortality attributable to hip fracture. These papers and a published systematic review by Abrahamsen et al.178 were examined to identify suitable data to include in the model.
The systematic review by Abrahamsen et al.178 examined the relationship between hip fracture and mortality, and found that patients with hip fracture experience a high mortality rate, which is at least double that for age-matched population norms. Abrahamsen et al.178 also noted that while the highest excess risk appears to be in the first 6 months following fracture, many of the studies they examined found an increased risk that persisted for a number of years. Age and sex were both found to be important predictors of post-fracture mortality supporting the use of age- and sex-specific estimates within our model.
Although there is clear evidence of excess mortality following hip fracture compared with general population norms, the extent to which underlying conditions contribute to the excess mortality associated with hip fracture is unclear.178 Underlying health conditions, which may be more common in patients experiencing hip fracture than in age- and sex-matched population norms, may contribute to mortality independently of the fracture itself, confounding the relationship between fracture and mortality. Kanis et al.179 found that 17–32% of deaths following hip fracture were causally related to fracture, whereas Parker and Anand180 estimated that 25% of deaths were directly attributable to hip fracture, with a further 42% possibly attributable to hip fracture. A study by Tosteson et al.,181 which was able to adjust for a number of prognostic factors including pre-fracture health status, found that excess mortality was limited to the first 6 months after fracture.
To populate the model, data were needed on the absolute risk of mortality following hip fracture that is directly related to the hip fracture and, therefore, potentially avoidable by treatment to prevent fractures. Age- and sex-specific estimates were sought because these are important risk-modifying factors identified in the systematic review by Abrahamsen et al.178 UK estimates were also considered preferable, as these are more likely to be representative of the population likely to be affected by NICE guidance. Of the studies included in the review by Abrahamsen et al.,178 10 reported results for UK cohorts.180,182–190 The majority of these studies do not report data on the absolute risk stratified by age and sex. Holt et al.187 provide graphs of survival at 120 days for different sexes and age bands. Deakin et al.183 provide age- but not sex-specific estimates of mortality at 30 days and 1 year rates. Parker and Anand180 provide age-specific mortality rates, but these are not reported separately for males and females. Only one study, by Roberts and Goldacre,189 provides age- and sex-specific mortality rates, and these are provided at 30, 60 and 365 days. This study used data from the Oxford record linkage study,191 which comprises anonymised abstracts of hospital statistics linked to death certificates. The population examined by Roberts and Goldacre189 was 32,590 people aged ≥ 65 years who were admitted to hospital as emergencies with fractured neck of femur between 1968 and 1998. Mortality rates were compared over six time windows between 1968 and 1998 and absolute mortality rates are provided for the cohort admitted with fractures between 1984 and 1998.
The studies included in the review of published cost-effectiveness analyses were also examined to determine the source of data used. Stevenson et al.140 used unpublished estimates from the Anglian audit of hip fracture, which were reported for several different age bands, and adjusted these to remove those deaths not causally related to hip fracture using the data from Parker and Anand.140,180 Ström et al.,139 Borgström et al.137 and Kanis et al.142 used data from Sweden179,192,193 rather than data from the UK. van Staa et al.141 estimated excess mortality rates from a UK database of general practice patients (GPRD, which is now called CPRD) and absolute rates are presented by age band, but this cohort was restricted to postmenopausal women. A Cox proportional hazards model was used by van Staa et al.141 to compare 1-year mortality rates for those with fracture and controls without fracture, who were matched based on age, GP practice and calendar time. Similar methods were used in another of the included cost-effectiveness papers, by van Staa et al.,143 which identified cases and controls from the same UK database but examined a population treated with steroids. However, this paper did not report the absolute mortality risks calculated. No additional studies were identified from the papers by Kanis et al.138 and Borgström et al.146
The age- and sex-specific mortality rates reported by Roberts and Goldacre189 for 1 year were much higher than the excess rates reported by van Staa et al.141 This is to be expected because the estimates from van Staa et al.141 are the excess mortality rates compared with age- and sex-matched controls, whereas the estimates reported by Roberts and Goldacre189 are raw mortality rates. As our aim was to include only the excess mortality associated with hip fracture in our model. The rates reported by van Staa et al.141 were incorporated in the model for women in preference over the data from Roberts and Goldacre.189 The excess rates in men were estimated by applying the ratio of raw events observed between men and women from Roberts and Goldacre189 to the excess rates for women from van Staa et al.141
The excess mortality rates attributable to hip fracture that have been applied in the model are presented in Table 19. In the PSA, these rates have been varied by estimating the numbers in each category in the patient cohort used by van Staa et al.141 by assuming that the age distribution is similar to that of the general population159 and using the estimated number with and without excess mortality to inform a beta distribution for each age band. The ratio of excess mortality rates for males versus females was not varied in the PSA.
TABLE 19
Excess mortality rates attributable to hip fracture
Abrahamsen et al.178 report that approximately half of all mortality associated with hip fracture occurred within 3 months and 70% occurred by 6 months. Given that Tosteson et al.181 reported no excess mortality after 6 months following adjustment for a variety of factors, including pre-fracture functional status and comorbid conditions, we decided to assume that all deaths related to hip fracture occurred at exactly 3 months. A sensitivity analysis was conducted examining the alternative assumption that all deaths related to hip fracture occurred at exactly 1 month post fracture. Hip fractures occurring before age 50 years were assumed not to result in any excess mortality.
A systematic review by Smith et al.157 found that the RR of death following hip fracture for those residing at home compared with those residing in an institution prior to hip fracture was 0.57 (95% CI 0.43 to 0.72) when meta-analysed across five studies including a total of 25,497 participants. To reflect the increased risk of mortality for those institutionalised prior to hip fracture, we applied a RR of 1.75 (1/0.57) to the figures in Table 19 for those residing in institutional care prior to hip fracture. This may have slightly overestimated the risk of mortality following hip fracture, as some of the patients included in the study by van Staa et al.141 will have been institutionalised and, therefore, the risks for non-institutionalised patients should be adjusted down. However, the study by van Staa et al.141 does not report the proportion institutionalised by age category within their sample so this adjustment was not possible. The likely bias introduced by not adjusting these figures is expected to be small, as the majority of patients within the model do not reside in institutional care (see Figure 70).
Mortality after vertebral fracture
The approach used to model mortality after vertebral fracture is similar to that used to model mortality after hip fracture. All of the papers included in the review of published cost-effectiveness analyses included some estimate of mortality following vertebral fracture within their economic evaluation. These papers were examined to determine the source data used.
The cost-effectiveness analysis by van Staa et al.141 used estimates of mortality following clinical vertebral fracture which were derived by the authors themselves from a UK cohort of postmenopausal women identified from a database of general practice patients (GPRD). The methods used in this paper to estimate mortality after vertebral fracture were the same as those used to estimate mortality after hip fracture and have been described above in Mortality after hip fracture. Excess mortality rates are presented in this paper by age band, but are limited to women. As described previously in Systematic review of existing cost-effectiveness evidence, a second paper by van Staa et al.143 used a similar method to estimate excess mortality after fracture in a cohort of UK patients treated with steroids but mortality rates were not reported in this second paper.
Two of the included cost-effectiveness papers137,139 reported using estimates from Oden et al.,193 but the absolute mortality rates could not be identified from the cited paper. Kanis et al.142 cited seven studies192,194–199 that provide data on the mortality risk after vertebral fracture. The only study to use a UK cohort, by Jalava et al.,197 examined the impact of prevalent and incident vertebral fractures on mortality rates in patients enrolled in a RCT of clodronic acid. Jalava et al.197 commented that the small size of this study’s cohort limited its ability to detect a mortality effect related to incident fractures with only seven deaths occurring in patients with incident vertebral fractures.197 Kanis et al.142 used the RR associated with prevalent vertebral fractures from the UK study by Jalava et al.197 to determine the rate of deaths associated with vertebral fractures in their cost-effectiveness model. Data from a Swedish study by Kanis et al.199 were used by Kanis et al.142 to determine the percentage of deaths (28%) that were causally related to vertebral fracture and data from a second Swedish study by Johnell et al.192 were used to justify applying the same RR for males and females. Kanis et al.199 provide estimates of the absolute risk of mortality stratified by sex and age bands and adjusts this to account for the proportion of deaths that are causally related. Johnell et al.192 provide estimates of excess absolute risks by sex for ages 60 and 80 years by comparing the mortality rate in those with fractures against age- and sex-matched general population controls. The remaining studies cited by Kanis et al.142 did not provide estimates of absolute risk stratified by age and sex. No additional studies were identified from the cost-effectiveness studies by Kanis et al.,138 Borgström et al.146 and Stevenson et al.140
It should be noted that not all of the studies identified agreed about the causal nature of the relationship between vertebral fractures and mortality. Several studies found no statistically significant increase in mortality rates for incident fractures after adjusting for potential confounding factors.197,200 Those studies that found a significant relationship192,195,196,199 often did not adjust for potential confounding factors other than age and sex, although Cauley et al.194 did find a significant increase after adjusting for six comorbidities and pre-fracture health status.
Differences between findings across studies may also be related to whether or not they considered morphometric vertebral fractures or only those coming to clinical attention, which are likely to be more severe. The study by Kanis et al.199 considered only hospitalised vertebral fractures, which could be expected to be more severe and associated with a higher death rate than non-hospitalised clinical vertebral fractures.
Some studies used baseline radiographs to confirm that the incident fracture was in fact new and not an undiagnosed prevalent fracture,194,200 but many studies195,196,199 assumed that fractures that came to clinical attention had occurred recently. Kado et al.198 considered only the impact of prevalent vertebral fractures on mortality. The impact of incident fractures on mortality for the same cohort were considered in a later publication by Kado et al.200 Those studies that considered morphometric fractures may also be complicated by the potential for delay between the fracture and the time it is found on a radiograph. Kado et al.,200 whose study relied on a single radiograph during the follow-up period to identify incident morphometric fractures, noted that some fractures might have occurred between the last radiograph and the end of follow-up, with those patients being allocated to the no-fracture group.
The data reported by van Staa et al.141 were used in the model, as this study used a large UK cohort, adjusted for multiple confounding factors and reported the excess risk for incident clinically symptomatic vertebral fractures. Although Center et al.195 reported higher standardised mortality rates for men than for women when considering all vertebral fractures, the differences were small when considering incident vertebral fractures alone [1.6 (95% CI 1.4 to 1.8) in women vs. 1.8 (95% CI 1.6 to 2.0) in men]. Johnell et al.192 reported a non-significant trend for a higher RR in men than women and Kanis et al.199 noted that the difference was not marked after taking into account sex differences in mortality within the general population. Therefore, we used the excess rates for women from van Staa et al.141 and applied these to both men and women within our model. The timing of excess mortality attributable to vertebral fracture was less well discussed in the identified studies than for similar data for hip fracture. However, a graph of death hazard over time for both hip and vertebral fractures, presented by Kanis et al.,199 suggests that a similar temporal pattern is seen for hip and vertebral fracture with high excess mortality in the early months. Therefore, we assumed that all mortality related to vertebral fracture occurred at 3 months, as this was the assumption used for hip fracture-related mortality.
The excess rates following vertebral fracture applied in the model are presented in Table 20.
TABLE 20
Excess mortality rates following vertebral fracture
In the PSA, the parameter uncertainty around these excess mortality rates has been calculated using the same method used for excess mortality following hip fracture (see Mortality after hip fracture).
Excess mortality risk at fracture sites other than hip or vertebrae
Patients experiencing fractures at sites other than the hip or vertebrae were assumed not to experience a fracture-related death. Having examined the published cost-effectiveness analyses to identify evidence on the increased risk of mortality following fractures at other sites, we decided that the evidence was not sufficient to support including this in the model.
Three of the seven papers included in our review of published cost-effectiveness analyses included an increased mortality risk for fractures at the proximal humerus.140,142,143 Two of these studies140,142 cited the paper by Johnell et al.,192 which found an increased risk of mortality compared with age- and sex-specific matched general population estimates among patients with shoulder fracture, although the increase was not statistically significant at all ages. The third paper, by van Staa et al.,143 used Cox proportional hazards models to assess the excess mortality in the year following hip, wrist, vertebral and proximal humerus fractures in a population treated with steroids compared with age- and sex-matched control subjects. These 1-year excess risks were incorporated in their analysis for all four fracture sites, but no data on the excess risks are presented in the paper. In a similar analysis, van Staa et al.141 examined the excess mortality associated with hip, wrist, vertebral and proximal humerus fracture in a UK population of postmenopausal women. However, they found that the excess risk of mortality was small for fracture types other than hip or vertebral fracture and they did not include any estimates of excess mortality for wrist or proximal humerus fractures in their analysis in postmenopausal women. A study by Cauley et al.,194 which analysed mortality rates before and after fracture using data from a RCT, found no increased risk of mortality for fractures at sites other than the hip or vertebrae after adjusting for six comorbidities and pre-fracture health status. However, a more recent paper by Piirtola et al.201 found that the mortality rates following proximal humerus fractures were significantly increased in men but not women. Given that the evidence for an excess risk of mortality following proximal humerus fracture is not consistent across the studies we examined, we have not included any increased mortality risk for proximal humerus fractures.
Only one of the published cost-effectiveness analyses included in our literature review incorporated an increased risk of mortality for wrist fractures.143 This paper used estimates derived by the authors of a general practice database for a cohort treated with steroids, but estimates of the excess mortality by fracture type were not provided in the paper.143 However, two of the published analyses140,142 stated that their assumption of no increased mortality risk following wrist fractures was consistent with published surveys.192,194–196 We have assumed no increased risk of mortality following wrist fracture in our analysis.
Stevenson et al.140 and Kanis et al.142 grouped fractures occurring at sites other than the hip, wrist, proximal humerus and vertebrae into one of these four fracture types. This meant that the excess mortality of hip fracture was also attributed to femoral shaft and pelvic fracture, and the excess mortality for proximal humerus fractures was also attributed to fractures of the humeral shaft, tibia and fibula. In our model, we have grouped other femoral fractures, but not pelvic fractures, with hip fractures so that the excess mortality risk associated with hip fracture is also applied to other femoral fractures. The data we have used on excess mortality following hip fracture were taken from the paper by van Staa et al.,141 which also grouped other femoral factures with hip fractures and, therefore, the data are being used in a manner consistent with what they were intended for. In summary, our analysis allows for excess mortality following fractures at the hip, femoral shaft or vertebrae but not for any other fracture site.
Risk of nursing home admission following hip fracture
Pain, reduced physical function and lack of mobility are common outcomes after hip fracture and can lead a patient who was previously living independently to require long-term nursing care. Patients experiencing a hip fracture who are community dwelling prior to fracture are assumed in the model to be at risk of moving to a nursing home or residential care. The risk is applied as a one-off risk at the time of the fracture event. The published economic analyses described in Systematic review of existing cost-effectiveness evidence were examined to identify sources of data on this parameter and this was supplemented by a scoping search to find additional sources.
All of the published cost-effectiveness studies included in our review appeared to include some estimate of nursing home admission within their model. Two studies138,139 included in the review of published cost-effectiveness analyses cited a conference poster by Zethraeus et al.,202 which gives the proportion of patients going into long-term care in the year following hip fracture surgery in Sweden by age band. Two of the published studies140,142 used data from the East Anglian hip audit.203 Three of the studies included in the review of published cost-effectiveness analyses137,141,143 cited a report describing the model, which was later published by Stevenson et al.140 as their source of data on nursing home admission following hip fracture, suggesting that they too applied the data from the East Anglian hip audit.203
As the only UK data identified from the published cost-effectiveness analyses were data from a 1999 research report,203 more recent data were sought to inform the risk within the model of patients moving from living in their own home to nursing home care after hip fracture. Age- and sex-specific data were sought, as it was believed that there may be a differential risk according to the age and sex of the patient. A scoping search identified a small number of papers addressing the issue of nursing home admission after hip fracture, of which four contained data on risk of discharge by both age and sex.158,204–206 These papers are summarised in Table 21.
TABLE 21
Summary of studies identified reporting risk of discharge to nursing home care after hip fracture by age and sex
The study by Holt et al.,204 despite covering a large sample in a UK population, was excluded on the basis that the analysis by age included only two age groups with relatively wide bounds (50–64 years and 75–89 years) and excluded patients aged 65–74 years. This was thought inadequate to assess the increasing risk of nursing home discharge with age.
We calculated approximate age- and sex-specific probabilities for discharge to a non-home location using the overall probability of discharge to institutional care and ORs for age and sex reported by the remaining three studies.158,205,206 Studies by Osnes et al.158 and Nanjayan et al.206 gave similar results, but Osnes et al.158 was thought less appropriate to the UK setting because of the potential for differences in social care structure and cultural norms regarding institutional care between the UK and Norway. Of the two UK studies, the study by Nanjayan et al.206 was preferred because the analysis explicitly excluded those who had died before discharge and was based solely on patients who were living in their own home prior to the fracture. Both of these criteria matched the model requirements, and hence data from Nanjayan et al.206 were used in preference to those from Deakin et al.205 (Figure 86).

FIGURE 86
Comparison of calculateda discharge to non-home location rate by age for two UK, and one Norwegian study. Values based on Nanjayan et al. F, females; M, males. a, Calculated based on ORs reported in the studies.
The overall rate of discharge to a non-home location (residential home, nursing home or hospitalisation) was given as 20% by Nanjayan et al.206 Combining this with the known sex split of the cohort (71% female) and the stated ORs for each age and sex group, it was possible to derive an expected risk of non-home discharge for each age and sex group for use in the model; these are shown in Table 22. The risk of being discharged to a non-home location increases with increasing age (OR 9.09 for patients aged between 90 and 99 years whereas OR = 1 for patients of approximately 69 years) and is higher for males than for females (OR 1.67).
TABLE 22
Rate of new admission to an institutional residential setting, calculated from age- and sex-specific ORs
The risks of a new admission to an institutional residential setting after hip fracture, presented in Table 22, have been applied within the model. In the PSA, these have been varied by applying a beta distribution to the overall rate of admission to an institutional residential setting on which the rates in the individual age and sex categories is dependent (see Appendix 8, Table 53, for details on PSA distributions).
Risk of nursing home admission following vertebral fracture
In the base-case analysis we have assumed that vertebral fractures do not result in new admissions to nursing homes or residential care. The published economic evaluations identified in Systematic review of existing cost-effectiveness evidence were examined to identify sources of data on nursing home admission following fracture. Only one of the papers142 included in our review of published cost-effectiveness analyses included a rate of nursing home admission following vertebral fracture. Kanis et al.142 incorporated data on the rate of nursing home admissions in Swedish patients from a paper by Borgström et al.,207 which reported similar rates of patients living in ‘special living accommodation’ for hip and vertebral fractures. However, Borgström et al.207 also noted in their discussion that, in their patient sample, the proportion hospitalised was higher than expected (72% vs. expected 10%). The study by Borgström et al.207 recruited patients at the time of fracture and no comparison was made with matched control subjects to remove costs that may be related to comorbidities. In comparison, a study, by De Laet et al.,208 that did compare costs in fracture patients and matched control subjects found that nursing homes costs were substantially higher for hip fracture patients than for control subjects, but were only slightly and non-significantly higher for vertebral fracture patients. However, this analysis, conducted as part of the Rotterdam study,209 included patients with a new morphometric fractures and may therefore underestimate resource use in those with clinically apparent vertebral fractures. Given the lack of consensus on the incorporation of nursing home admission rates within the published analyses and the differing data from these two studies, we decided to omit nursing home admission following vertebral fracture from our base-case model, but examined the impact of including a rate equivalent to that seen in hip fracture in a sensitivity analysis.
Risk of subsequent fracture after incident fracture
A systematic review and meta-analysis by Klotzbuecher et al.149 has previously been used in several published economic evaluations to estimate the increased risk of fracture at various sites when a patient sustains an incident fracture within the model.139,140,142 We conducted a citation search, using the Web of Science database, to find relevant articles published since the review by Klotzbuecher et al.,149 on the assumption that new studies in this area would be likely to cite this published systematic review. We found 811 records of articles citing this systematic review. Given the large number of potentially relevant articles identified, we tried to establish whether or not any more recent systematic reviews had been published. The abstracts and titles of these articles were then searched separately using the free-text terms ‘review’, ‘meta-analysis’ and ‘synthesis’ to see if any of these articles provided an updated systematic review and meta-analysis similar to that presented by Klotzbuecher et al.149 Two potential systematic reviews were identified and full texts examined.210,211 The first, by Haentjens et al.,210 was specifically interested in comparing whether or not the RR of hip fracture after a wrist or spine fracture differed by sex. Owing to its focus on sex differences, this study had narrower inclusion criteria and excluded many of the studies included by Klotzbuecher et al.149 and it only included one additional recent study.212
The second systematic review identified from our citation search, which was authored by Blank (on behalf of the FRAX Position Development Conference Members),211 identified around 20 studies published since the Klotzbuecher et al.149 review. However, these studies are discussed narratively by Blank and no meta-analysis is provided.211 It was not considered feasible to review and meta-analyse all of these new studies in order to update the estimates provided by Klotzbuecher et al.149
A 2011 review by Warriner et al.,213 which meta-analysed data from 25 studies published since the Klotzbuecher et al.149 review, was identified opportunistically. The review by Warriner et al.213 does not provide any details regarding the methods used to identify the studies. It also provides limited details on the studies included and does not tabulate the RRs from the individual studies prior to pooling. Therefore, it was decided that the estimates from Warriner et al.213 should be treated with caution because of the potential for selection bias. The estimates provided by Klotzbuecher et al.149 were used in the base-case model. These estimates were supplemented by data from Warriner et al.213 where no estimates were provided by Klotzbuecher et al.149 Neither meta-analysis provided data on the increased risks of fracture following proximal humerus fracture. Data on the increased risk following fracture at any site were used as a proxy for risk following fractures at the proximal humerus. Neither meta-analysis provided data on the risk of proximal humerus fracture after hip fracture, so the data on proximal humerus fracture following fracture at any site from Warriner et al.213 were used. The data in Table 23 were applied in the model as HRs within the survival curves, used to estimate time to fracture for the base-case analysis. A sensitivity analysis has also been conducted using the estimates from Warriner et al.213 exclusively, which are shown in Table 24.
TABLE 23
Increased risk of subsequent fracture following incident fracture
TABLE 24
Increased risk of subsequent fracture following incident fracture used in sensitivity analysis
The values from Klotzbuecher et al.149 and Warriner et al.213 are applied for the patient’s remaining lifetime once a fracture occurs. The studies included by Klotzbuecher et al.149 in the meta-analysis had varying durations of follow-up, but generally > 1 year, so the estimates provided by Klotzbuecher et al.149 represent the RR when averaged over all years of study follow-up. The temporal profile of increased fracture risk after an incident fracture has been studied by van Geel et al.214 Their analysis suggests that the RR is approximately 2 when averaged over the long term, but when assessed over different time periods is much higher immediately after the first fracture, and tails off towards 1 over the next 20 years. We acknowledge that our method of applying a fixed RR over the patient’s remaining lifetime probably underestimates the increased risk in the immediate years after fracture, but it is likely to overestimate the increased risk in the long term. The alternative would be to use additional dummy events to modify the increased risk in the years after fracture, but this would reduce the computational efficiency of the model. In the PSA, the HRs in Table 23 were sampled from a log-normal distribution using standard errors calculated from the 95% CIs reported in Table 23 (see Appendix 8, Table 52, for PSA distributions).
When more than one incident fracture was sampled to occur during a patient’s lifetime, the maximum value from Table 23 has been applied for each subsequent fracture type rather than applying several multipliers concurrently. For example, if someone has had a prior wrist fracture and a prior vertebral fracture then their increased risk of vertebral fracture is 4.4, which relates to their prior history of vertebral fracture, as this is the maximum value in the vertebral column in Table 23. However, their increased risk for proximal humerus fracture would be 2.5, which relates to their prior history of wrist fracture, as this is the maximum value in the proximal humerus column.
Both QFracture and FRAX incorporate an increased risk for patients with a history of prior fracture and, therefore, those with a prior fracture at the start of the model already have an increased risk applied for prevalent fractures. This increased risk associated with fractures occurring prior to the start of the model is removed at the time of the first incident fracture and the data from Table 24 are applied instead. This is to prevent the risk being increased twice for the same patient characteristic using two different mechanisms within the model.
Health-related quality of life: review of utility values following fracture
To inform the model, data were needed on the proportionate decrease in HRQoL that occurs in the year following fracture and in subsequent years. This was then used to calculate a utility multiplier, which was applied to the pre-fracture utility value to calculate the post-fracture utility. For example, a proportionate decrease of 10% would translate into a utility multiplier of 0.9. If the patient’s prior fracture utility is 0.8, then the post-fracture utility would be 0.72. Data on the absolute HRQoL after fracture can be obtained from studies that measure HRQoL in patients who have experienced a recent fracture. However, the proportionate decrease can be obtained only if there is some estimate of pre-fracture utility. Ideally, HRQoL would be measured prospectively in a cohort of patients at risk of fracture and these patients would be followed up with HRQoL remeasured at regular intervals with the time of any incident fracture being recorded so that the correlation between HRQoL and incident fracture can be obtained after adjusting for other confounding factors. However, many studies simply recruit patients at the time of fracture and ask them to recall their pre-fracture health state, which is subject to recall bias. Other studies may compare the HRQoL in individuals who have fractured with matched controls or population norms, in which case the estimates may be confounded by differences in other factors between cases and controls.
Initially, a systematic search was conducted to identify studies reporting any measure of health utility in patients with an incident osteoporotic fracture. However, this search retrieved 3991 unique references and it was not considered feasible to sift such a large number of papers within the timescales of the NICE appraisal process. As the NICE methods guide144 states that the EQ-5D is the preferred measure of HRQoL in adults, and a recent systematic review by Peasgood et al.215 had already demonstrated that EQ-5D data exist for the four major osteoporotic fracture sites, the search was made more specific, with the aim of identifying only those studies reporting HRQoL data measured using the EQ-5D. This more sensitive search retrieved 132 references and was sifted for relevant papers.
Studies reporting HRQoL values measured during RCTs were excluded because of the possibility that study interventions may affect HRQoL independently of their impact on fracture. In addition, studies that examined the HRQoL impact of surgical interventions to treat fracture were excluded as these were focused on comparing the impact of different surgical techniques on quality of life rather than comparing pre- and post-fracture HRQoL under usual management. Studies reporting the quality-of-life impact of prevalent fractures were excluded on the basis that there is no way of knowing how long ago the prevalent fracture was sustained and the model requires information on the quality-of-life impact in the year following fracture and in subsequent years.
Sixteen studies remained207,216–230 (summarised in Table 25), of which eight provided HRQoL for hip/fractures,216,219,220,224–227,230 eight for wrist fractures,216,217,220,221,226–228,230 10 for vertebral fractures207,216,217,220,222,226,227,229,230 and two for shoulder fractures.217,220 Of these, two studies used non-UK utility values216,217 and two were of very specific patient cohorts,217,218 making the results of these studies less relevant to the general population at risk of fragility fracture. Cooper et al.218 focused on women with inadequate response to therapy and Ekström et al.219 focused on patients with subtrochanteric hip fractures only. Therefore, HRQoL values from these studies were not considered further.
TABLE 25
Summary of included papers reporting EQ-5D quality-of-life measures associated with osteoporotic fracture
Four studies did not provide a pre-fracture or control utility value220–223 and these were excluded except where no other values were available.
Five of the included papers contained duplicate results,207,224–227 as both papers by Tidermark et al.224,225 referred to the same study and the papers by Ström et al.226 and Borgström et al.227 referred to a single study [known as KOFOR (the costs and effects of osteoporosis-related fractures study)]. The later paper by Borgström et al.227 was an international extension to the KOFOR study [known as ICUROS (the International Costs and Utilities Related to Osteoporotic fractures Study)] which gave HRQoL values by country but not pooled values. The Swedish cohort within ICUROS appeared to have been based on a slightly expanded version of the KOFOR sample. Of the ICUROS results, the Swedish values were thought to be the most appropriate because they were based on the largest sample of the various country-specific cohorts and they were expected to provide a good estimator of UK HRQoL values, as northern European countries have been shown to have similar values.231
Values from eight papers207,224–230 reporting outcomes from five distinct studies were therefore compared. All studies appeared to observe similar patterns in HRQoL, with an immediate, severe drop in HRQoL associated with the acute fracture incident (where recorded), followed by a recovery to a higher HRQoL within the first 4 months, and stabilisation or slow improvement over the course of the year to 12 months. The exception to this was the Roux et al.230 study, which was a prospective study in which utility was measured at enrolment (pre fracture) and then after 12 months, with the post-fracture values being 12-month values for patients who experienced a fracture at any time during the previous 12 months. As a result, values from the Roux et al.230 study showed a gradual decline over a 12-month period. The advantage of this approach is that pre-fracture utilities were as measured and, therefore, not subject to recall bias. Values at 12 months should also theoretically represent an average of utility loss associated with fracture over a year, assuming all patients were surveyed at exactly 12 months. However, as a significant amount of utility loss is experienced in the first days and weeks after fracture, the results could easily be biased if patients who had recently experienced a fracture delayed completing the survey. As the study was based on self-completion postal questionnaires, it was considered possible that there may be some reporting bias in this study and, therefore, values from other studies were considered more appropriate. One of the papers by Tidermark et al.225 did not report a HRQoL value between baseline and 4 months and, therefore, this study did not observe the severe drop in HRQoL associated with the acute fracture incident. A summary of the values reported by individual studies for utility after hip fracture, wrist fracture, vertebral fracture and shoulder fracture are presented in Tables 26–29, respectively.
TABLE 26
Utility values after hip fracture
TABLE 29
Utility values after shoulder fracture
TABLE 27
Utility values after wrist fracture
TABLE 28
Utility values after vertebral fracture
Values were plotted and a weighted average score was calculated for each fracture type. An example is shown in Figure 87 for hip fracture, for which five appropriate papers were sourced,207,224–227 relating to two studies. The weighted average score closely followed the result of the largest study (KOFOR/ICUROS) reported in the papers by Ström et al.226 and Borgström et al.207 Similar patterns were observed for all fracture types. The KOFOR/ICUROS study was the only study to provide pre- and post-fracture values for hip, wrist and spine fractures. It also had the largest sample size and reported similar results to other studies. Therefore, the decision was made to use values from the KOFOR/ICUROS study as the basis of the utility multipliers applied in the model. However, no study provided complete HRQoL data for shoulder fracture, so in this case values from Zethraeus et al.220 were used, with an assumption that post-fracture HRQoL measured at 12 months represented a return to pre-fracture HRQoL levels. No studies reported pre-fracture (or control) and post-fracture values for fractures at sites other than the hip, wrist, spine or shoulder.

FIGURE 87
Illustration of post-fracture trends in HRQoL following hip fracture taken from five papers reporting on two different studies plus a weighted average.
The average utility value in the first year following fracture has been calculated by assuming an immediate drop in HRQoL at fracture maintained for 1 month, followed by a linear improvement to 4 months and then a further linear improvement to 12 months. The utility multiplier applied in the first year post fracture was then calculated as the ratio of the average utility in the year post fracture to the baseline utility prior to fracture. The utility value observed at 12 months is assumed to persist in the long term, so the multiplier for the second and subsequent years was set to the ratio of the 12-month and pre-fracture utility value.
The data applied in the model are summarised in Table 30. The post-fracture utility values have been varied in the PSA by sampling values from a beta distribution (see Table 51, Appendix 8, for details on the distributions).
TABLE 30
Calculation of utility multipliers from quality-of-life study results
Health-related quality-of-life values for institutionalisation
The published economic analyses described in Systematic review of existing cost-effectiveness evidence were examined to identify sources of data on HRQoL for patients living in nursing home or residential care compared with those dwelling in the community. Additionally, a paper by Tidermark et al.225 containing relevant data was opportunistically identified from the systematic review of papers reporting HRQoL following fracture.
Tidermark et al.225 found that, in a prospective cohort study of 90 patients with hip fracture who were living independently prior to their fracture, patients with an independent living status at 4 months after fracture had significantly higher EQ-5D scores than those living in institutions (0.64 and 0.35, respectively; p < 0.05). A similar difference in mean scores (0.56 vs. 0.35) was seen at final follow-up (> 12 months after fracture with mean follow-up of 17 months), but this was no longer statistically significant. The lack of statistical significance at final follow-up may be because of the small number of patients institutionalised (seven at 4 months and eight at 17 months). We used the data from the final follow-up within our analysis to calculate a utility multiplier for nursing home admissions following fracture of 0.625. This is higher than the value of 0.4 used in four of the published analyses;140–143 however, this earlier value was based on judgement by an expert panel.153 The remaining three137–139 published analyses did not describe the utility multiplier applied for nursing home admission. The multiplier calculated from Tidermark et al.225 was used in our model, as this was based on EQ-5D scores valued using the UK tariff, which is consistent with the NICE reference case.144 Tidermark et al.225 did not report SDs for the mean EQ-5D values for institutionalised patients and patients living independently. To provide an estimate of uncertainty in the utility multiplier within the PSA, the standard error around the utility multiplier was set to give a 95% CI that coincided with no difference between these two health states, to reflect the lack of a statistically significant difference in the mean values at 17 months.
Age- and sex-specific utility values in the absence of clinical events
Utility in patients without fracture is dependent on age and sex and is based on EQ-5D data for the UK general population.232 The age- and sex-dependent utility value applied to the period between two events is taken to be the average of the utility at the start and end of that period. This ensures that patients who do not experience any events do not stay at an artificially high level of utility, equivalent to the utility value for their age at the start of the model. The regression used to calculate utility from age and sex is as follows:
where sex is 1 for males and 0 for females, and age is in years.
A multivariate normal distribution, which takes into account the correlation between the regression coefficients, was used to sample the regression coefficients in the PSA.
Resource use and costs for bisphosphonates treatment
Drug costs for oral bisphosphonates have been taken from the The Electronic Drug Tariff,151 as it is assumed that these are prescribed in primary care.144 zoledronic acid and i.v. ibandronic acid are assumed to be prescribed in secondary care and costs for these have therefore been taken from the electronic market information tool (eMIT) database, which reports the average cost paid by secondary care trusts for generic medicines.144,152 It was noted by our clinical advisors that generic zoledronic acid has only recently become available and, therefore, the prices reported by the eMIT database may be higher than those currently being paid in the NHS, as the price is likely to fall after a generic preparation becomes available and the current eMIT database uses data from the 12 months prior to June 2014. Therefore, a sensitivity analysis was conducted using the price for the 4-mg preparation of zoledronic acid, which is for a different indication but has been available in generic form for a longer time. This was felt to represent a realistic lower limit for the price of the 5-mg zoledronic acid preparation.
Where there was more than one preparation available we have assumed that the lowest-cost preparation is prescribed based on the average cost for 1 year of treatment. Therefore, for alendronic acid and risedronic acid we assumed that weekly preparations are prescribed, as these had the lowest costs based on the The Electronic Drug Tariff151 costs applied in the model; these are summarised in Table 31. Drug prices are assumed to be known precisely and, therefore, have been assumed to be fixed within the PSA.
TABLE 31
Costs based on the The Electronic Drug Tariff
Oral therapies were assumed to incur no additional costs for administration. The costs of i.v. administration of zoledronic acid and ibandronic acid have been based on NHS reference costs.150 ibandronic acid is given by i.v. injection over 15–30 seconds. It is assumed that this is done during an outpatient endocrinology consultation at a cost of £133 (NHS reference cost £302).150 zoledronic acid is given by i.v. infusion over a longer duration and this is assumed to be done as a day case. The reference cost for a day case delivery of a simple parenteral chemotherapy (SB12Z at £245)150 has been applied, as no alternative reference costs were identified which would cover day case admissions for the administration of a drug by infusion. The outpatient cost for the same HRG code (SB12Z) is £165, suggesting that it is classification of this activity as a day case rather than the specific nature of chemotherapy that makes this more expensive than an outpatient endocrinology appointment. It was therefore considered reasonable to apply the day case reference cost for parenteral chemotherapy as a proxy for the cost of delivering zoledronic acid owing to the longer duration of administration compared with i.v. ibandronic acid. Our clinical advisors noted that in some cases zoledronic acid is administered as an outpatient procedure and, therefore, a sensitivity analysis was conducted using the outpatient cost for both i.v. bisphosphonates. Reference costs for the administration of i.v. bisphosphonates were varied in the PSA (see Table 63, Appendix 8 for details).
Resource use and costs of fracture
The published economic analyses were searched to identify published evidence on resource use attributable to fracture. This was supplemented by ad hoc searches for UK studies published since 2006, as this was when a DSU report detailing several different methods to estimate fracture costs was published.174
Resource use attributable to fracture was based on a UK study by Gutierrez et al.,233,234 which used a GP database (The Health Improvement Network) to estimate resource use for those who fractured compared with matched controls. Patients were matched on age, GP practice and comorbidity score. The study was reported in two separate papers, with the first reporting the costs attributable to hip fracture233 and the second reporting the costs attributable to vertebral fracture, non-hip non-vertebral fracture and also some less detailed results for wrist and proximal humerus fracture.234 The study examined hospitalisations, accident and emergency (A&E) visits, referrals, prescriptions and GP contacts in the year following fracture. It did not examine any costs falling within PSS, such as nursing home admission or home help. The authors also noted that they did not include rehabilitation costs, but they did estimate the total cost including rehabilitation by using estimates of rehabilitation costs from other published studies.
The difference in the percentage of patients using each type of resource between those who had fractured and matched controls was multiplied by the unit cost to get the average cost per fracture in the year following fracture. Unit costs for hospitalisations, A&E appointments and specialist referrals were based on NHS reference costs, while unit costs for social care and GP appointments were based on estimates from the PSSRU. Tables 32 and 33 show the difference in resource use between patients who fractured and their matched controls and the unit costs applied. The total first year and subsequent year costs are summarised in Table 34. Unit costs for A&E vary by fracture type as different costs were applied for admitted and non-admitted patients and these proportions vary by fracture type. Unit costs for prescriptions were calculated by dividing the difference in total prescription cost by the difference in the mean number of prescriptions using data from Gutierrez et al.234 However, this detailed information was not available for wrist and proximal humerus fractures, so data from the broader category of non-hip non-vertebral fractures were used for wrist and proximal humerus.
TABLE 32
Resource use attributable to fracture
TABLE 33
Unit costs for resource use attributable to fracture
TABLE 34
Summary of fracture costs in the year following fracture and in subsequent years
In the cost-effectiveness analysis that informed TA16021 and TA161,24 it was assumed that patients who experienced a vertebral fracture had ongoing costs in the second and subsequent years associated with the long-term prescribing of treatments to manage the chronic symptoms associated with vertebral fractures. The analysis by Gutierrez et al.234 does not examine costs beyond the first year; however, it can be seen that for vertebral fracture, non-hip non-vertebral fractures and hip fractures, the costs of medications are fairly stable in the first and second 6 months following fracture, whereas the costs for health-care contacts such as GP appointments, referrals and A&E visits fall sharply in the second 6 months. Therefore, we decided to apply prescription costs as an ongoing cost from the time of fracture. All other costs estimated by Gutierrez et al.234 were applied in the first year only.
In the analysis by Stevenson et al.,140 Swedish data presented by Borgström et al.207 were used to estimate the costs of home help. We used the same data on the average number of hours of home help following fracture, as used by Stevenson et al.,140 but applied present-day unit costs. Home help costs are assumed to occur in only the first year after fracture and apply only to those residing in the community and not to institutionalised patients.
For patients living in an institutional residential setting we applied the cost of local authority-provided residential care for older people with the unit cost (£1100 per week) taken from the PSSRU.26 The costs for local authority-provided care were used instead of private sector or NHS residential care, as a recent report by The King’s Fund235 states that the vast majority (78%) of residential care places are provided by local authorities. We assumed that 36% of patients self-fund their residential care based on data presented by the Care Quality Commission.236 The annual cost falling within the NHS and PSS budget was therefore estimated at £36,608 per person in residential care per annum. In the PSA, both the resource-use estimates in Table 32 and the unit costs taken from NHS reference costs were sampled from probabilistic distributions. Those taken from PSSRU were not varied in the PSA as the PSSRU does not report a measure of variance. Further details on the distributions used in the PSA are provided in Appendix 8, Table 51.
The costs for each of the four main osteoporotic fracture sites have been applied to other sites in the same grouping (e.g. other femoral has the same cost as hip).
Approach to sensitivity analysis
A PSA has been conducted to estimate the mean cost and QALYs gained when taking into account the uncertainty in the parameter values used within the model. In general, parameters were estimated using the following distributions: gamma distributions for costs; log-normal distributions for HRs; and beta distributions for utility values and probabilities. None of the parameters used to estimate fracture risk, in the absence of treatment, was varied in the PSA. This was to ensure that a specific set of patient characteristics was consistently mapped to the same survival curve for fracture-free survival without any parameter uncertainty. The following additional parameters were not varied in the PSA: drug prices; discount rates; unit costs sourced from PSSRU; utility in the second year after proximal humerus fracture; life expectancy after fracture associated with excess mortality; unit costs for prescriptions after fracture; and proportion of self-funders for residential care. Full details on the distributions applied within the model can be found in Appendix 8, Tables 51–62.
Structural sensitivity analyses were conducted to explore whether or not the results were sensitive to different model assumptions. In the majority of cases these were conducted using the deterministic model, which does not incorporate any parameter uncertainty, owing to the significant computational time required to run the PSA. A summary of the structural sensitivity analyses conducted is provided in Table 35 alongside an indication of the model used.
TABLE 35
Summary of structural sensitivity analyses
Results
Patient characteristics of the simulated cohort
Summary patient characteristics are provided in Table 36 for each risk category when using both FRAX and QFracture to calculate the absolute fracture risk. It can be seen that the average age is higher in the higher-risk categories and the proportion of patients with the risk factors of prior fracture, steroid use or nursing home residency increases in the higher-risk categories. The proportion of women also appears to increase in the higher-risk categories as would be expected given that women in general have a higher risk of osteoporotic fracture than men.
TABLE 36
Summary patient characteristics for each risk category defined by either FRAX or QFracture deciles
It should be noted that, in addition to there being different risk cut-off points for the risk categories when using either QFracture or FRAX scores to define absolute risk, the ranking of patients by risk within the cohort will differ between the two algorithms. It is therefore possible that patients falling into a particular risk category when using the QFracture algorithm may fall into a different risk category when using the FRAX algorithm. Figure 88 shows the distribution of 200,000 patients eligible for risk assessment under CG14616 across the QFracture and FRAX risk categories. It can be seen from Figure 88 that, although there is some agreement over the categorisation of patients across the two risk scoring algorithms, there is not perfect agreement. The correlation between the absolute risk scores was found to be 0.83 and the correlation between the risk categories based on deciles of risk score was found to be 0.76.

FIGURE 88
Distribution of patients across FRAX and QFracture risk categories. QFracture risk categories are indexed Q1–10 and FRAX risk categories are indexed F1–10, with 1 being the lowest risk category in each case.
Clinical outcomes predicted by the model
Clinical outcomes for 200,000 patients are presented in Table 37 for the base-case scenario in which we have applied the mean persistence with treatment from observational data. Under these assumptions the numbers needed to treat to prevent one fracture during the first 6 months (6 months being the duration of persistence with oral bisphosphonates) is lowest for risedronic acid and highest for oral ibandronic acid. Given that it is necessary to treat around 2000 patients to prevent one fracture during the period of persistence with oral bisphosphonates treatment when using the QFracture risk score, we estimated that we would need to simulate approximately 2 million patients to obtain stable estimates of the benefits of treatment in each risk category. This is because we would expect around 1000 fractures to be prevented across a cohort of 2 million patients, with around 1% falling within the lowest risk category of QFracture. Therefore, the costs and QALY implications of treatment would be based on around 10 fractures in the lowest risk category of QFracture when using a cohort of 2 million patients.
TABLE 37
Clinical outcomes for 200,000 patients when applying mean persistence from observational studies
It can be seen from Table 37 that the number of fractures occurring in the first 6 months is higher when using the FRAX algorithm than when using the QFracture algorithm. This is because the absolute risk predicted by FRAX is higher than the absolute risk predicted by QFracture in 98% of patients.
Presentation of cost-effectiveness results
The mean costs and QALYs from the PSA are presented as the base-case results. These were considered to be preferable to estimates obtained using mid-point (mean or median) parameter inputs because we believe that there may be a non-linear relationship between parameter values and model outcomes. The data presented were obtained from a total patient population of 2 million across all 10 risk categories, with one parameter sample per patient. Therefore, approximately 200,000 patients and 200,000 parameter samples informed the estimates for each risk category.
Full results tables for the base-case scenario including an incremental analysis for each risk category for QFracture and FRAX are presented in Tables 64–73 in Appendix 9 and Tables 74–83 in Appendix 10, respectively. Results have been summarised below by plotting the incremental net benefit (INB) compared with a strategy of no treatment when assuming that a QALY is valued at £20,000. INB has been plotted instead of ICERs, as these can be difficult to interpret when the QALY gain is negative, which was the case for some treatments in some risk categories. The cost-effectiveness plane has not been presented, as a minimum of 20 graphs would be needed to present results across all 10 risk categories for both QFracture and FRAX. We used non-parametric regression to estimate the cost-effectiveness acceptability curves (CEACs). This allows variation in the costs and QALYs as a result of parameter uncertainty to be separated from variation caused by patient-level stochastic variability.
Structural sensitivity analyses have been conducted by fixing parameter values at their mid-point value. Although it would have been preferable to rerun the PSA for each structural sensitivity analysis, this was not possible within the time constraints. The PSA was rerun for the sensitivity analysis, which involved changing the HRs for treatment as we considered it important in this case to capture the underlying joint distribution for the HRs. For the sensitivity analyses on AE rates and the sensitivity analysis examining alternative treatment costs for zoledronic acid, the outputs of the base-case PSA model were adjusted, as these adjustments could be made without rerunning the PSA. For all other sensitivity analyses, the model using mid-point parameter estimates was run for 2.2 million patients.
Summary of cost-effectiveness results for the base-case scenario when using QFracture
Figure 89 summarises the cost-effectiveness results across the 10 risk categories when using QFracture to estimate absolute risk. It shows the INB, in monetary terms, when valuing a QALY at £20,000, when compared with a strategy of no treatment. Each point shows the mean INB and the mean 10-year absolute risk of fracture for one risk category for a particular bisphosphonate treatment. It can be seen that the mean INB is close to zero for all three oral bisphosphonates across the first six risk categories, which have mean absolute risks ranging from 0.5% to 2.7%; the estimates are all very close together.

FIGURE 89
Incremental net benefit (when valuing QALY at £20,000) compared with no treatment against the 10-year fracture risk from QFracture.
Detailed results tables providing a full incremental analysis are provided in Tables 64–73 in Appendix 9. It can be seen from these that in the third, fourth and sixth risk categories (mean absolute risks of 1.0%, 1.4% and 2.7%, respectively) at least one of the oral bisphosphonates has a positive INB, but the absolute INB is still small and close to zero. In the fifth risk category (mean absolute risk of 2%) it is below zero for all three oral bisphosphonates. The INB is positive for all three oral bisphosphonates from the seventh to the tenth risk categories (mean absolute risk of 3.9% and above). A strategy of no treatment has the maximum net benefit in the first, second and fifth risk categories (mean absolute risks of 0.5%, 0.7% and 2.0%, respectively) and when a QALY is valued at either £20,000 or £30,000 (see Tables 64–72 in Appendix 9 for INB at £30,000). In the other risk categories the treatment with maximum net benefit is always either alendronic acid or risedronic acid. Oral ibandronic acid does not fall on the cost-effectiveness frontier in any risk category when using QFracture to estimate absolute risk. The difference between oral ibandronic acid and the other two oral bisphosphonates becomes more apparent in the higher-risk categories. This is because of marginally less favourable efficacy data for oral ibandronic acid, which becomes more important as the risk increases. For the i.v. bisphosphonates the INB is negative across all 10 risk categories when valuing a QALY at either £20,000 or £30,000 (see Tables 64–73 in Appendix 9 for INB at £30,000).
The full data from the PSA for the whole population (2 million patients with one parameter sample per patient) were used in a non-parametric regression, which estimated the relationship between INB and absolute fracture risk estimated by QFracture. For each of the five treatment options, the relationship between the INB of treatment (vs. no treatment) and the absolute fracture risk was estimated using a generalised additive model. This flexible regression approach assumes that the underlying relationship between the dependent variable and the independent variable can be approximated by a cubic spline, a highly flexible function that can model any smooth relationship between two variables. The regression prediction is shown in Figure 90 with a close-up provided in Figure 91 of the lower-risk range. The results here differ from those presented in Figure 89 because the non-parametric regression method is able to average over the stochastic uncertainty associated with the individual-level patients while simultaneously estimating the relationship between INB and absolute risk. It can be seen that the INB of alendronic acid and risedronic acid increases with increasing risk. A strategy of no treatment is predicted to have the greatest net benefit for the lowest-risk patients. Table 38 summarises the thresholds over which each treatment has a positive INB compared with no treatment (when valuing a QALY at £20,000) and the range over which each treatment has the maximum INB based on the non-parametric regression. Alendronic acid is predicted to have the maximum net benefit from 1.5% and risedronic acid is predicted to have the maximum net benefit from 7.2% upwards. Oral and i.v. ibandronic acid have differing relationships with absolute risk, which may reflect the fact that different efficacy data were applied. However, the results for i.v. ibandronic acid should be treated with caution as no fracture data were available for this treatment and data from other ibandronic acid regimens were applied. It should also be noted that the regression may predict INB less well in higher-risk patients, as only 10% of the population had a risk score of > 11%. It is also important to consider the uncertainty around the INB estimates by considering the CEACs.

FIGURE 90
Regression for INB (when valuing QALY at £20,000) compared with no treatment against the 10-year fracture risk from QFracture.

FIGURE 91
Close-up of regression for INB (when valuing QALY at £20,000) compared with no treatment against the 10-year fracture risk from QFracture.
TABLE 38
QFracture absolute risk thresholds obtained from regression of INB compared with no treatment over absolute risk (when valuing a QALY at £20,000)
Figures 92–101 present the CEACs for each of the risk categories when using QFracture to determine absolute risk. It can be seen that in the first and second risk categories (mean absolute risk of 0.5% and 0.7%, respectively), the no-treatment strategy has a much higher probability of being optimal, when valuing a QALY at £20,000 than any of the other strategies. However, in the third risk category (mean absolute risk of 1.0%), no treatment has the third highest probability of being most cost-effective with both risedronic acid and oral ibandronic acid having a greater probability when valuing a QALY at either £20,000 or £30,000. Although all three oral bisphosphonates have a positive INB compared with no treatment, in the seventh risk category (mean absolute risk of 3.9%) when valuing a QALY at £20,000, no treatment has a higher probability of being cost-effective than either risedronic acid or oral ibandronic acid, suggesting that there is still considerable uncertainty regarding the relative cost-effectiveness of oral bisphosphonates.

FIGURE 92
Cost-effectiveness acceptability curve for QFracture risk category 1 (mean absolute risk of 0.5%).

FIGURE 101
Cost-effectiveness acceptability curve for QFracture risk category 10 (mean absolute risk of 16.0%).

FIGURE 93
Cost-effectiveness acceptability curve for QFracture risk category 2 (mean absolute risk of 0.7%).

FIGURE 94
Cost-effectiveness acceptability curve for QFracture risk category 3 (mean absolute risk of 1.0%).

FIGURE 95
Cost-effectiveness acceptability curve for QFracture risk category 4 (mean absolute risk of 1.4%).

FIGURE 96
Cost-effectiveness acceptability curve for QFracture risk category 5 (mean absolute risk of 2.0%).

FIGURE 97
Cost-effectiveness acceptability curve for QFracture risk category 6 (mean absolute risk of 2.7%).

FIGURE 98
Cost-effectiveness acceptability curve for QFracture risk category 7 (mean absolute risk of 3.9%).

FIGURE 99
Cost-effectiveness acceptability curve for QFracture risk category 8 (mean absolute risk of 5.5%).

FIGURE 100
Cost-effectiveness acceptability curve for QFracture risk category 9 (mean absolute risk of 8.4%).
The i.v. bisphosphonates have a low probability of being optimal when valuing a QALY at £20,000, even in the highest-risk categories, although by the 10th risk category (mean absolute risk of 16.0%) they have a similar probability of being cost-effective as no treatment.
Summary of cost-effectiveness results for the base-case scenario when using FRAX
Figure 102 summarises the cost-effectiveness results across the 10 risk categories for FRAX. It shows the INB for each bisphosphonate treatment when compared with no treatment plotted against the 10-year absolute risk of fracture. Each point shows the mean INB and the mean 10-year absolute risk of fracture for one risk category when valuing a QALY at £20,000. It can be seen that the INB compared with no treatment does not have a simple relationship with absolute risk when using FRAX to define absolute risk. At first, the INB rises, but it later falls before rising again. This may reflect the differing patient characteristics across the risk categories. However, it can be seen that the mean INB compared with no treatment is above zero for all oral bisphosphonates across all 10 risk categories. The detailed results tables provided in Appendix 9 show that none of the bisphosphonates is consistently more cost-effective than the others, with all three having the highest INB (when valuing a QALY at £20,000) in at least one risk category and all three being dominated by another oral bisphosphonate in at least one risk category.

FIGURE 102
Incremental net benefit (when valuing QALY at £20,000) compared with no treatment against the 10-year fracture risk from FRAX.
The mean INB for the two i.v. bisphosphonates is below zero across all 10 risk categories. This remains the case even when valuing a QALY at £30,000 (see Tables 74–83 in Appendix 10). Furthermore, i.v. ibandronic acid is always extendedly dominated by the other treatment strategies across all 10 risk categories for FRAX.
The full data from the PSA for the whole population (2 million patients with one parameter sample per patient) were used in a non-parametric regression, which estimated the relationship between INB and absolute fracture risk estimated by FRAX. For each of the five treatment options, the relationship between the INB of treatment (vs. no treatment) and the absolute fracture risk was estimated using a generalised additive model. This flexible regression approach assumes that the underlying relationship between the dependent variable and the independent variable can be approximated by a cubic spline, a highly flexible function that can model any smooth relationship between two variables. The regression prediction is shown in Figure 103, with a close-up shown in Figure 104 for the lower risk range. The results here differ from those presented in Figure 102 because non-parametric regression is able to average over the stochastic uncertainty associated with the individual-level patients while simultaneously estimating the relationship between INB and absolute risk. It can be seen that the INB of alendronic acid and risedronic acid increases with increasing risk. For all three oral bisphosphonates, the INB is positive compared with no treatment across the full range of absolute risk observed in the modelled population. Table 39 summarises the thresholds over which the INB of each treatment is positive compared with no treatment (when valuing a QALY at £20,000) and the range over which the INB of each treatment is maximum based on the non-parametric regression. Oral ibandronic acid is predicted to have the maximum INB up to an absolute risk level of 8.6%. Alendronic acid is predicted to have the maximum net benefit from 8.6%% to 38.5% and risedronic acid is predicted to have the maximum net benefit from 38.5% upwards. The INB compared with no treatment is negative for both the i.v. bisphosphonates across the full range of absolute risk observed in the modelled population when using FRAX to estimate absolute risk. By comparing Figure 90 with Figure 103 it can be seen that the relationship between INB and absolute risk for the i.v. bisphosphonates appears to differ when using FRAX and QFracture for patients with an absolute risk of > 20%. However, this may not reflect a true difference, as the estimates of > 11% for QFracture and > 18% for FRAX are informed by only one-tenth of the modelled population and, therefore, it is also important to consider the uncertainty in these estimates of mean INB by considering the CEACs.

FIGURE 103
Regression for INB (when valuing QALY at £20,000) compared with no treatment against the 10-year fracture risk from FRAX.

FIGURE 104
Close-up of regression for INB (when valuing QALY at £20,000) compared with no treatment against the 10-year fracture risk from FRAX.
TABLE 39
FRAX absolute risk thresholds obtained from regression of INB compared with no treatment over absolute risk (when valuing a QALY at £20,000)
Figure 105–114 show the CEACs for the 10 FRAX risk categories. It can be seen that the strategy of no treatment has a low probability of being most cost-effective when valuing a QALY at £20,000, across all 10 risk categories. The i.v. bisphosphonates always have a lower probability of being optimal compared with no treatment or the oral bisphosphonates until risk category 8 (mean absolute risk of 10.7%) when i.v. zoledronic acid has a higher probability of being cost-effective than no treatment. In FRAX risk category 10 (mean absolute risk of 25.1%), i.v. zoledronic acid has the highest probability of being cost-effective, when valuing a QALY at £20,000 and i.v. ibandronic acid has a higher probability than oral ibandronic acid. However, it should be noted that the mean INB for both the i.v. bisphosphonates is negative in this risk category when valuing a QALY at £20,000.

FIGURE 105
Cost-effectiveness acceptability curve for FRAX risk category 1 (mean absolute risk of 3.1%).

FIGURE 114
Cost-effectiveness acceptability curve for FRAX risk category 10 (mean absolute risk of 25.1%).

FIGURE 106
Cost-effectiveness acceptability curve for FRAX risk category 2 (mean absolute risk of 4.3%).

FIGURE 107
Cost-effectiveness acceptability curve for FRAX risk category 3 (mean absolute risk of 5.0%).

FIGURE 108
Cost-effectiveness acceptability curve for FRAX risk category 4 (mean absolute risk of 5.6%).

FIGURE 109
Cost-effectiveness acceptability curve for FRAX risk category 5 (mean absolute risk of 6.2%).

FIGURE 110
Cost-effectiveness acceptability curve for FRAX risk category 6 (mean absolute risk of 7.3%).

FIGURE 111
Cost-effectiveness acceptability curve for FRAX risk category 7 (mean absolute risk of 8.8%).

FIGURE 112
Cost-effectiveness acceptability curve for FRAX risk category 8 (mean absolute risk of 10.7%.)

FIGURE 113
Cost-effectiveness acceptability curve for FRAX risk category 9 (mean absolute risk of 14.9%).
Summary of cost-effectiveness results for the base-case scenario when using FRAX
Figure 115 summarises the results from the model using mid-point parameter inputs. It shows the INB for each bisphosphonate treatment when compared with no treatment plotted against the 10-year absolute risk of fracture. The ‘F’ and ‘Q’ labels after the drug name indicate where the FRAX and QFracture algorithms have predicted the risk, respectively. The INB at the various risk levels appear to fall on a slightly higher curve when using FRAX than when using QFracture, with the difference being more pronounced for the i.v. bisphosphonates. This behaviour was also observed when examining the PSA results for QFracture and FRAX on the same plot, but the difference was slightly less pronounced (data not presented).

FIGURE 115
Incremental net benefit for the base-case scenario when using mid-point parameter estimates. F, predicted by FRAX; Q, predicted by QFracture.
Structural sensitivity analyses
A sensitivity analysis was conducted in which we assumed that all patients would persist with treatment for the intended treatment duration (5 years for oral bisphosphonates and i.v. ibandronic acid and 3 years for zoledronic acid). In Figure 116, it can be seen that the INB is positive for oral bisphosphonates in all but the lowest risk category when using QFracture and in all risk categories when using FRAX. This is to be expected because the absolute benefits of treatment are greater when assuming that patients persist with treatment for longer. Therefore, as treatment continues the net benefit of treatment outweighs the upfront costs and disutilities associated with AEs in the first month after initiating treatment. The ICER for i.v. ibandronic acid compared with no treatment falls below £30,000 per QALY in the eighth risk category for FRAX (mean absolute risk of 10.7%) and below £20,000 per QALY in the 10th risk category of FRAX (mean absolute risk of 25.1%). For QFracture, the ICER compared with no treatment for i.v. ibandronic acid remains above £30,000 per QALY across all risk categories. For zoledronic acid, the ICER compared with no treatment does not fall below £30,000 in any risk category for either FRAX or QFracture.

FIGURE 116
Incremental net benefit for the sensitivity analysis assuming full persistence with treatment for 3 years for zoledronic acid and 5 years for all other bisphosphonate treatments. F, predicted by FRAX; Q, predicted by QFracture.
A sensitivity analysis was conducted in which the rate of admission to a nursing home following hip fracture was applied to both hip and vertebral fractures. The results for this analysis are presented in Figure 117. The results are broadly similar to the base-case results, suggesting that our decision not to include nursing home admission following vertebral fracture within the analysis is unlikely to have significantly biased the cost-effectiveness results.

FIGURE 117
Incremental net benefit for sensitivity analysis applying nursing home admission rates following hip fracture to vertebral fractures in addition to hip fractures. F, predicted by FRAX; Q, predicted by QFracture.
A sensitivity analysis was conducted in which we removed any fractures occurring at sites other than the four main osteoporotic fracture sites (hip, wrist, proximal humerus and vertebrae). The INBs compared with no treatment for both QFracture and FRAX are summarised in Figure 118. It can be seen that the results when using the QFracture algorithm are similar to the base case, but the results when using the FRAX algorithm have a lower INB and are more closely aligned with those for QFracture when considering risk categories with a similar mean absolute risk. The results from this structural sensitivity analysis suggests that the method used to uplift the fracture risk to incorporate fractures at additional sites may have caused the INBs of bisphosphonate treatment to be overestimated in the base-case analysis based on FRAX.

FIGURE 118
Incremental net benefit for the sensitivity analysis excluding fractures occurring at sites other than the hip, wrist, proximal humerus and vertebrae. F, predicted by FRAX; Q, predicted by QFracture.
A sensitivity analysis was conducted in which the survival curves for hip fracture were based on the hip-specific absolute risk estimates from QFracture rather than a proportion of the absolute risk for the four main osteoporotic fracture sites. The results, shown in Figure 119, are broadly similar to the base case although the INB estimates for the FRAX risk categories are generally lower and fall closer to those for the QFracture categories with comparable absolute fracture risk. The INBs for all three oral bisphosphonates are negative in the first FRAX risk category (mean absolute risk of 3.1%) and the INB for risedronic acid is negative in the second FRAX risk category (mean absolute risk of 4.3%). The results of this structural sensitivity analysis suggests that the base-case scenario may have overestimated the cost-effectiveness of treatment for the FRAX risk categories because of the method used to calculate survival curves for FRAX from the data available for QFracture. The cost-effectiveness results for bisphosphonates treatment compared with no treatment may therefore be favourable to treatment when using the FRAX risk scores.

FIGURE 119
Incremental net benefit for scenario using hip-specific estimates of absolute fracture risk. F, predicted by FRAX; Q, predicted by QFracture.
In the analysis, assuming full persistence with treatment, the duration of treatment for zoledronic acid was 3 years but the fall-off period was set to 7 years, while for the other bisphosphonates the treatment duration was 5 years and the fall-off period was 5 years. Although the assumption ensured that treatment effects fell to zero at 10 years for all drugs, when assuming full persistence, this assumption might have be favourable to zoledronic acid. In the base-case scenario in which mean persistence from observational studies was applied, the treatment duration and fall-off period for zoledronic acid were set to 1.7 years and 4 years (7/3 x 1.7), respectively. A sensitivity analysis was conducted in which the fall-off period for zoledronic acid was set equal to the treatment duration (1.7 years for both). The results are summarised in Figure 120. It can be seen that for lower-risk categories for QFracture the INB estimates for zoledronic acid do not vary smoothly suggesting that they have failed to reach a stable estimate probably owing to the limited number of fractures prevented when assuming only 1.7 years of treatment and 1.7 years of fall-off time. However, the INB for zoledronic acid versus no treatment remains below zero for all risk categories for both QFracture and FRAX, as was observed in the base-case scenario.

FIGURE 120
Incremental net benefit for scenario in which fall-off time was set equal to treatment duration for zoledronic acid. F, predicted by FRAX; Q, predicted by QFracture.
A sensitivity analysis was conducted to examine whether or not uncertainty regarding the average survival in patients who die following a hip fracture was an important determinant of cost-effectiveness. For this analysis the average duration of survival after hip fractures associated with excess mortality was reduced from 3 months to 1 month. The results, which are summarised in Figure 121, are very close to those seen in the base-case scenario and, therefore, it can be concluded that the exact duration of survival following a hip fracture associated with excess mortality is not an important determinant of cost-effectiveness.

FIGURE 121
Incremental net benefit when assuming that excess mortality associated with hip fractures occurs 1 month after the hip fracture. F, predicted by FRAX; Q, predicted by QFracture.
A sensitivity analysis was conducted using the more recent data on the increased risk of fracture following an incident fracture from the systematic review by Warriner et al.213 The results, summarised in Figure 122, show marginally higher INBs for treatment compared with no treatment, which is expected because several of the HRs for increased fracture risk following an incident fracture were greater in the paper by Warriner et al.213 than the figures presented in the paper by Klotzbuecher et al.149 which were used in the base-case scenario. However, the results do not appear to be particularly sensitive to the choice of data source for these model parameters.

FIGURE 122
Incremental net benefit for sensitivity analysis using Warriner et al. instead of Klotzbuecher et al. as the preferred source for the HR of subsequent fracture following incidence fracture. F, predicted by FRAX; Q, predicted by QFracture.
A sensitivity analysis was conducted in which the prevalence of a prior fracture at baseline was estimated from UK fracture incidence data rather than using estimates of the prevalence of a prior fracture from a meta-analysis of the cohort studies which informed the FRAX algorithm. It can be seen from Figure 123 that the results are very similar to the base-case results and, therefore, the model is not particularly sensitive to the prevalence of a prior fracture at baseline. This may be because a history of prior fracture has only a marginal impact on the individual’s utility and health resource use and the increased risk attributed to prior fracture would simply move patients between risk categories rather than making it more or less cost-effective to treat within a particular risk category.

FIGURE 123
Incremental net benefit for sensitivity analysis using UK incidence data to estimate the prevalence of prior fracture. F, predicted by FRAX; Q, predicted by QFracture.
In the base-case analysis, data from the 150 mg per month of oral ibandronic acid regimen were applied in the model for the monthly oral dose for all four fracture sites. However, no fracture data were available for the i.v. ibandronic acid regimen. As this regimen was licensed based on a non-inferiority trial comparing it to the previously licensed 2.5 mg per day oral regimen, data from the 2.5-mg oral dose were applied to the i.v. regimen where these were available. Where these were not available, data from the 150 mg per month oral regimen were applied instead. However, this meant that different data were applied for the oral and i.v. regimen for some fracture sites (vertebral and proximal humerus). A sensitivity analysis was conducted in which the same efficacy data were applied to both the monthly oral and the quarterly i.v. ibandronic acid regimens. For vertebral and proximal humerus fractures data from the 2.5 mg per day oral ibandronic acid regimen were applied to both as both were licensed based on non-inferiority trials comparing them to the daily 2.5-mg oral dose (no longer licensed). Data for hip and wrist were unchanged as the only data available were for the 150-mg dose and these data were applied to both regimens in the base case. The efficacy data applied in the base-case and the sensitivity analysis are summarised in Table 40.
TABLE 40
Hazard ratios applied in the base-case and sensitivity analysis for ibandronic acid treatment regimens
The results for this sensitivity are summarised in Figure 124 for the QFracture risk categories and in Figure 125 for the FRAX risk categories. The estimates presented here are the mean outputs from the PSA, which incorporated the joint distribution of the HRs from the NMA. The results are very similar to the base-case analysis, suggesting that the model is not particularly sensitive to the choice of data source for the ibandronic acid HRs. This was to be expected given that the NMA did not find any strong evidence of a difference in efficacy between the monthly and daily dosing ibandronic acid regimens. It remains possible that there is a difference between fracture outcome for the monthly oral and quarterly i.v. regimens, but this could not be assessed within the NMA because no fracture outcomes were available for the quarterly i.v. regimen.

FIGURE 124
Incremental net benefit for sensitivity analysis using the same efficacy data for oral and i.v. ibandronic acid treatments for QFracture risk categories.

FIGURE 125
Incremental net benefit for sensitivity analysis using the same efficacy data for oral and i.v. ibandronic acid treatments for FRAX risk categories.
Scenario sensitivity analyses were also conducted on the costs and QALY decrements attributable to AEs. As AEs were not included as an uncertain parameter in the PSA, it was possible to adjust the PSA outputs for different assumptions regarding AEs. Figures 126 and 127 summarise the results when assuming no costs or QALY decrements attributable to AEs for the QFracture and FRAX risk categories, respectively. It can be seen that in this scenario the oral bisphosphonates are more cost-effective, with only risedronic acid having a negative INB compared with no treatment in the first QFracture risk decile (mean absolute risk of 0.5%), when valuing a QALY at £20,000. In all other risk categories the oral bisphosphonates have a positive INB, except for the fifth risk category (mean absolute risk of 2.0%), in which only alendronic acid has a positive INB. However, the results for the i.v. bisphosphonates are similar, with negative INBs compared with no treatment across all 10 risk categories for QFracture.

FIGURE 126
Incremental net benefit for sensitivity analysis assuming no costs or QALY decrements for adverse side effects for QFracture risk categories.

FIGURE 127
Incremental net benefit for sensitivity analysis assuming no costs or QALY decrements for adverse side effects for FRAX risk categories.
The results across the FRAX risk categories when assuming no costs of QALY decrements attributable to AEs were similar to the base-case scenario, with positive INBs for the oral bisphosphonates and negative INBs for the i.v. bisphosphonates when valuing a QALY at either £20,000 or £30,000.
In addition, a scenario analysis was conducted in which the rate of adverse side effects for oral bisphosphonates was increased from 3% to 30%. In this scenario none of the oral bisphosphonates had a positive INB compared with no treatment across any of the QFracture risk categories when valuing a QALY at £20,000, as shown in Figure 128. The INBs remained negative for all treatments when valuing a QALY at £30,000 (data not presented).

FIGURE 128
Incremental net benefit for sensitivity analysis assuming a 30% AE rate for oral bisphosphonates in the first month of treatment for QFracture risk categories.
The results for the FRAX risk categories when assuming an AE rate of 30% for oral bisphosphonates in the first month of treatment are shown in Figure 129. It can be seen that the INB is negative for the three oral bisphosphonates for the first eight risk categories (mean absolute risk of 10.7% and below), but is positive for alendronic acid in the ninth FRAX risk category (mean absolute risk of 14.9%) and for all three oral bisphosphonates in the 10th FRAX risk category (mean absolute risk of 25.1%).

FIGURE 129
Incremental net benefit for sensitivity analysis assuming a 30% AE rate for oral bisphosphonates in the first month of treatment for FRAX risk categories.
Our clinical advisors were concerned that the price of zoledronic acid, which was taken from the eMIT database,152 may not be reflective of real-world prices because zoledronic acid has only recently become available in a generic format for this indication. Therefore, we conducted a sensitivity analysis using the price from eMIT for the 4-mg dose of generic zoledronic acid, which is licensed for the prevention of skeletal-related events in patients with advanced malignancies involving the bone. The average price on eMIT for the most commonly prescribed preparation of zoledronic acid for this alternative indication was £5.76. It was also noted by clinicians that zoledronic acid may be administered in some cases as an outpatient procedure rather than as a day case. Therefore, we also applied these lower administration costs in addition to the lower drug acquisition cost. This was done using the average outputs from the PSA and by assuming 1.67 doses of zoledronic acid are administered on average, with the mean number of doses estimated based on 500,000 PSA samples.
The results, when assuming these lower costs for zoledronic acid treatment, are summarised in Figure 130 for both QFracture and FRAX. It can be seen that, although the INB compared with no treatment has increased for zoledronic acid under these more favourable cost assumptions, the INB is still negative across all 10 risk categories for both QFracture and FRAX.

FIGURE 130
Incremental net benefit for zoledronic acid when assuming a lower acquisition price and outpatient rather than day case administration costs. F, predicted by FRAX; Q, predicted by QFracture.
Discussion
In summary, when valuing a QALY at £20,000, a strategy of no treatment is predicted to have the greatest net benefit for patients with a QFracture score of < 1.5%. Alendronic acid is predicted to have the maximum net benefit from 1.5% to 7.2% and risedronic acid is predicted to have the maximum net benefit for a score of ≥ 7.2%. However, the absolute costs and QALY gains are small in patients with low absolute risk and there is considerable uncertainty regarding whether or not no treatment is the optimal strategy until the QFracture score is approximately 5.5% (the mean absolute risk for the eighth risk category for QFracture).
The mean INBs for oral bisphosphonate treatment compared with no treatment were positive across all FRAX risk categories. However, in the base-case scenario the INBs of bisphosphonate treatments compared with no treatment were generally higher for FRAX than QFracture for risk categories with similar absolute fracture risk. We would expect from the way the model is structured that the threshold for cost-effective treatment would be broadly similar across the two risk scores. The results of two structural sensitivity analyses suggest that the base-case analysis may have overestimated the INB of bisphosphonate treatment compared with no treatment. Given this possible bias in the estimates generated by the model using the FRAX risk score, and our belief that the results should be broadly similar across the two risk scores, it would be reasonable to assume that the absolute risk threshold estimated in the QFracture model could be applied to patients whose score had been calculated using either QFracture or FRAX.
Intravenous bisphosphonates had much higher ICERs than no treatment. In the highest-risk categories the ICERs for i.v. ibandronic acid and zoledronic acid compared with oral bisphosphonates were > £50,000 per QALY, even though the base-case analysis assumed longer durations of persistence with i.v. bisphosphonates than with oral bisphosphonates. Although the mean INB compared with no treatment for i.v. ibandronic acid did become positive at very high levels of absolute risk when using QFracture, the results when using FRAX went in the opposite direction. This may be because of the small number of patients and parameter samples informing the estimates at high levels of absolute risk, which makes these estimates more uncertain.
The results appeared to be broadly similar when we conducted the following structural sensitivity analyses: applying the risk of nursing home admission following hip fracture to vertebral fractures; shorter duration of survival for hip fractures associated with excess mortality; alternative data source for increased risk of fracture following incident fracture; alternative data source for prevalence of prior fracture at baseline; using the same efficacy estimates for oral and i.v. ibandronic acid; reducing the acquisition and administration costs for zoledronic acid; and reducing the fall-off period for zoledronic acid. The results were broadly similar for QFracture but slightly less favourable for FRAX for the sensitivity analysis in which fractures at additional sites were removed from the model and for the sensitivity analysis using hip specific absolute risks to estimate time to hip fracture. The results were more favourable to treatment when assuming full persistence with treatment or when assuming no AEs. The sensitivity analysis examining an AE rate of 30% in the month following initiation of oral bisphosphonate therapy showed that the cost-effectiveness of oral bisphosphonate is very sensitive to the rate of AEs experienced.
The model’s estimates of cost-effectiveness are generalisable to patients eligible for risk assessment under CG146,16 as this is the population we have simulated. However, there are some groups with secondary osteoporosis who may be considered eligible for risk assessment under CG14616 who have not been explicitly simulated within our model. Patients at increased risk of fracture after receiving hormone treatments for breast and prostate cancer have not been explicitly simulated, although patients with the more general risk factor of ‘any cancer’ have been included in the simulated cohort. Patients at increased risk of fracture following untreated premature menopause have not been simulated but the prevalence of HRT usage in female patients has been taken into account within the simulated cohort. We might expect the cost-effectiveness in these groups to be similar to groups with other secondary causes of osteoporosis that have been explicitly modelled, such as steroid-induced osteoporosis, provided the groups who have not been explicitly modelled have an increased risk of fracture and similar life expectancy to other causes of secondary osteoporosis that have been modelled.
We have applied all-cause mortality data from the UK general population to the whole modelled cohort. This may overestimate the cost-effectiveness of treating patients who have higher mortality risks because of the presence of comorbidities and, therefore, the cost-effectiveness estimates may be less generalisable to groups with lower-than-average life expectancy.
One of the strengths of the patient-level simulation approach we have used is that we have been able to simulate how the distribution of patient characteristics, such as age, varies between different risk scores and how this influences the cost-effectiveness of treatment. However, the patient-level simulation approach used required a large number of patients to be simulated because of the scarcity of events in lower-risk populations. This made it difficult to accurately measure the incremental costs and QALYs associated with treatment in the lowest-risk categories when the treatment durations were reduced to reflect real-world persistence with treatment. However, we were able to use non-parametric regression to estimate the relationship between INB and absolute risk across the whole modelled cohort when averaging over both parameter uncertainty and the stochastic uncertainty associated with patient-level simulations. This made it possible to estimate the absolute risk at which the INB crosses zero for each treatment to a more accurate level than could be achieved by simply examining the INBs for each risk category.
Fracture risk prediction within the model is based on the risk predicted over time from the QFracture algorithm, but when validating the model we identified some internal inconsistencies within QFracture, which have implications for our model. The underlying survival function applied in QFracture for patients without any risk factors incorporates a hazard that increases over time. This makes sense, as the hazard for fracture is likely to increase as the patient ages. However, the 1-year risk of fracture predicted for a patient 5 years after their 50th birthday is higher than the 1-year risk of fracture predicted in the following year for a 55-year-old. We have assumed that the data points from the earlier years of the QFracture algorithm are likely to be more reliable than points from later years, when there may have been fewer patients with follow-up in the cohort used to derive the QFracture algorithm. Hippisley-Cox and Coupland18 report that the 2012 QFracture algorithm was based on approximately 23.6 million patient-years of follow-up in approximately 3.1 million patients, suggesting that the mean duration of follow-up was around 7.6 years. We would therefore expect the model predictions to be more robust when used to estimate fracture risk over 5 years than over 10 years. Therefore, we have resampled the patient’s fracture risk every time an event occurs and at 5 and 10 years after baseline in all patients. In doing so we have ensured that we have repeatedly sampled from the early part of the survival curve, which should be less uncertain as it is based on more patients from the QFracture database. This does, however, result in some model behaviour that goes against clinical expectations in that the hazard for an individual patient may be lower in the sixth year of the model than in the fourth year despite the increase in the patient’s age. Unfortunately, there is no way to correct this internal inconsistency while using QFracture as the basis for risk prediction within the model. Introducing more frequent events to update risk at annual intervals would minimise the impact of this internal inconsistency but it would significantly reduce the computational efficiency of the model and would not remove the inconsistency altogether. However, this issue is not expected to bias the estimates of cost-effectiveness as it has an equal impact across all treatment strategies.
Several assumptions had to be made to incorporate the FRAX algorithm within the model. First, the FRAX calculator does not provide estimates of the fracture risk for different time periods. Therefore, we assumed that the shape of the survival curve for fracture-free survival would be similar in FRAX and QFracture and applied a simple ratio to the rate parameter of the QFracture survival curve to generate time-to-event estimates for the FRAX model. The ratio was calculated to ensure that the time-to-event estimates for the FRAX model generated a survival curve with the 10-year risk predicted by the FRAX model. Second, the FRAX algorithm provides the estimate of fracture risk after taking into account the competing risk of mortality, whereas the QFracture algorithm does not incorporate any competing mortality risk. Therefore, we may have underestimated the fracture risk in the FRAX model by applying our own competing mortality hazard on top of that incorporated by FRAX.
The estimates of INB versus absolute fracture risk appear to fall on a slightly higher curve for all treatments when using FRAX than when using QFracture. This difference in the INBs estimated for QFracture and FRAX risk categories with similar levels of absolute fracture risk could have occurred, in part, because of the different risk scores selecting patients with different characteristics into certain risk categories. For example, in the sixth QFracture risk category, which has a mean risk of 2.7%, the proportion of patients with a prior fracture was 41.6%, whereas in the first FRAX risk category, which has a mean risk of 3.1%, the proportion with a prior fracture was 6.4%. As those with a prior fracture have a lower starting utility, it is more cost-effective to prevent future fractures in those without a history of prior fracture. This may explain why the sixth QFracture risk category had lower estimates of INB for bisphosphonate treatment than the first FRAX risk category, despite having similar fracture risks. Furthermore, the structural sensitivity analyses conducted on hip fracture risk and the uplift for fractures at additional sites suggest that the INB of treatment with bisphosphonates compared with a strategy of no treatment may have been overestimated in the base case because of the method used to calculate the survival curve for FRAX from the survival curve for QFracture. We suspect this is because we have assumed that the proportion of major osteoporotic fractures that occur at the hip is the same across both risk tools, but in fact the proportion is lower for FRAX than QFracture in 64% of the modelled population. This suggests that the number of hip fractures may have been overestimated in the model based on FRAX in the base-case analysis.
Our population was sampled taking into account the correlation between age and sex, and the risk factors of prior fracture, steroid use and nursing home residency. The relationship between age, sex and BMI was also incorporated. However, there are likely to be other correlations within the general population that we have not captured. This may mean that the mix of patient characteristics within each decile may not perfectly reflect the mix within each risk category for the population eligible for risk assessment. However, we have tried to capture the correlations between those factors likely to affect risk independently of the absolute risk of fracture, as these have the most potential to bias the estimates of cost-effectiveness.
The model does not allow for patients to move from community living to an institutional residential setting at any time other than following a fracture, which may overestimate the impact of fractures that result in residential care in patients who would have eventually moved into residential care for other reasons. However, the model does allow for patients to live in residential care or to have experienced a prior fracture before being treated with bisphosphonates. This avoids treatment benefits being overestimated in these groups.
The decision to group fractures occurring at additional sites (scapula, clavicle, sternum, rib, pelvis, humeral shaft and femoral shaft) with one of the four main osteoporotic sites (hip, wrist, proximal humerus, vertebral) may have over- or underestimated the impact of fractures at these additional sites if these fractures have different costs and QALY implications from the ones they have been grouped with. However, evidence on the resource-use and HRQoL impactions of fractures was focused on the four main fracture sites associated with osteoporosis, making it difficult to identify site-specific evidence on the consequences of fracture for fractures occurring at other sites.
One of the key limitations of our analysis is that we have assumed that all of the bisphosphonate treatment strategies are viable options for all patients within the population. This allowed us to run the model once for the whole population eligible for risk assessment and to determine a single absolute risk threshold for cost-effective intervention with each bisphosphonate. Applying a strict interpretation of the licensed indications for each bisphosphonate would have required running the analysis multiple times for different groups who have different treatment options, which was not feasible. Although incremental analyses are usually conducted over a set of potentially interchangeable treatments, in reality it is often the case that some of the cohort of patients who are eligible for one treatment would be contraindicated for another and allowances are made for this when interpreting the cost-effectiveness results. For example, it is possible to rank the treatments in order of decreasing net benefit and treat with the next most cost-effective treatment when the optimal treatment is contraindicated.
Another limitation of our analysis is that we have assumed equal treatment effectiveness across all patients eligible for risk assessment under CG146.16 There was no evidence of differential treatment effects with respect to sex and age. However, there was some heterogeneity in treatment effects between studies, suggesting that differential treatment effects according to study characteristics and the effect of treatment on femoral neck BMD depended on the baseline response.
The cost-effectiveness results for i.v. ibandronic acid should be treated with some caution as the fracture data used were taken from studies which used a daily dose of oral ibandronic acid. This was considered reasonable as i.v. ibandronic acid was licensed based on its non-inferiority in lumbar spine BMD outcomes when compared with the daily ibandronic acid treatment regimen. Any uncertainty regarding whether or not equivalent lumbar spine BMD outcomes do in fact translate into an equivalent reduction in fractures has not been captured within our assessment of model uncertainty.
Our estimates of the costs attributable to fracture do not include the costs of rehabilitation and may therefore underestimate the total cost. They do, however, include costs for home help and residential care which fall within the NHS and PSS perspective recommended in the methods guide.144
The way in which the DES has been implemented only allows for one acute utility multiplier to be applied at any one time. This may mean that the utility decrement in the year following a severe fracture may be underestimated if another less severe fracture occurs within a year. This may have marginally biased the cost-effectiveness analysis against treatment with bisphosphonates by underestimating the benefits of treatments that prevent hip and vertebral fractures, which have the greatest utility impact in the year following fracture in populations with a high risk of fractures at other sites. However, two events occurring in the same year is expected to be a rare outcome, particularly in lower-risk patients, so any bias is expected to be small.
The model is sensitive to the assumptions regarding AEs, particularly in the low-risk populations, in which the mean absolute cost savings and QALY gains are small. We have included AEs for oral bisphosphonates using the rates observed in prescription event monitoring studies. However, no significant difference in upper GI AEs was found in the placebo-controlled RCTs for oral bisphosphonates. It is unclear whether or not this is because the RCT population are not representative of the real-world population, which may be more likely to experience AEs, or if the apparent increased risk in real-world cohorts is confounded by other factors which are controlled for within a RCT.
Our analysis has used the FRAX calculator for patients with unknown BMD, as CG14616 recommends that patients should receive a BMD scan only if they are close to the treatment threshold and, therefore, the majority of patients are expected to receive treatment without a BMD scan. FRAX also provides an estimate of fracture risk in patients with known BMD. It is possible that the threshold for cost-effective treatment when using the version of the FRAX calculator developed for patients with known BMD may be slightly different if BMD is correlated with patient characteristics that affect risk independently of BMD. However, to properly ascertain whether or not the treatment thresholds would be different, we would need information on the relationships between BMD and a range of other risk factors such as age, sex, prior fracture and steroid use. Including BMD within the model without information on these relationships would simply shuffle patients with similar characteristics between the different risk groups. Although information is available on the relationship between BMD and some of these risk factors, such as age and BMI,237 adding additional but incomplete information on the relationship between the various risk factors and BMD may introduce an unintended bias in the estimates of cost-effectiveness. Given that both the QFracture and FRAX algorithm have been developed for use without BMD, the correlations between the risk factors included in these risk sores and BMD is already incorporated within the calculation of fracture risk. Therefore, we decided not to run the model using the FRAX algorithm for patients with known BMD.
Although the mean INBs for treatment with oral bisphosphonates are positive at low levels of absolute risk, it is important to note that the absolute costs and benefits are small and the no-treatment strategy has a reasonable probability of being optimal until the QFracture score is above approximately 5.5% (the mean absolute risk for the eighth risk category for QFracture). It is therefore possible that patients and clinicians may not consider treatment worthwhile in the lowest-risk patients even though it may be cost-effective.
- Assessment of cost-effectiveness - A systematic review and economic evaluation o...Assessment of cost-effectiveness - A systematic review and economic evaluation of bisphosphonates for the prevention of fragility fractures
Your browsing activity is empty.
Activity recording is turned off.
See more...